Intelligent Automation
The Appleton Greene Corporate Training Program (CTP) for Intelligent Automation is provided by Dr. Raina Certified Learning Provider (CLP). Program Specifications: Monthly cost USD$2,500.00; Monthly Workshops 6 hours; Monthly Support 4 hours; Program Duration 24 months; Program orders subject to ongoing availability.
Personal Profile
Dr Raina is an accomplished consultant with experience in business process automation and digital technologies. He received a Bachelor of Engineering degree in Electronics from Mangalore University (India), a Master of Science degree in Computer Studies (Artificial Intelligence) from Essex University (UK) and a PhD in Computer Science from Bristol University (UK). He has over 25 years of experience and runs a small successful consultancy established over 10 years ago. He specialises in creating business value leveraging technology innovations and best practice. He has provided technical leadership on a number of large and complex, multi-million-dollar transformation programmes involving business process automation.
Dr Raina has industry experience in a variety of sectors including healthcare, finance, public sector, utilities and FMCG. He has worked with multi-national companies based in Europe and the US. He regularly blogs on topics related to process automation and innovative technologies. He is co-inventor on a recent patent application related to adapting chatbots for the air transport industry.
To request further information about Dr. Raina through Appleton Greene, please Click Here.
(CLP) Programs
Appleton Greene corporate training programs are all process-driven. They are used as vehicles to implement tangible business processes within clients’ organizations, together with training, support and facilitation during the use of these processes. Corporate training programs are therefore implemented over a sustainable period of time, that is to say, between 1 year (incorporating 12 monthly workshops), and 4 years (incorporating 24 monthly workshops). Your program information guide will specify how long each program takes to complete. Each monthly workshop takes 6 hours to implement and can be undertaken either on the client’s premises, an Appleton Greene serviced office, or online via the internet. This enables clients to implement each part of their business process, before moving onto the next stage of the program and enables employees to plan their study time around their current work commitments. The result is far greater program benefit, over a more sustainable period of time and a significantly improved return on investment.
Appleton Greene uses standard and bespoke corporate training programs as vessels to transfer business process improvement knowledge into the heart of our clients’ organizations. Each individual program focuses upon the implementation of a specific business process, which enables clients to easily quantify their return on investment. There are hundreds of established Appleton Greene corporate training products now available to clients within customer services, e-business, finance, globalization, human resources, information technology, legal, management, marketing and production. It does not matter whether a client’s employees are located within one office, or an unlimited number of international offices, we can still bring them together to learn and implement specific business processes collectively. Our approach to global localization enables us to provide clients with a truly international service with that all important personal touch. Appleton Greene corporate training programs can be provided virtually or locally and they are all unique in that they individually focus upon a specific business function. All (CLP) programs are implemented over a sustainable period of time, usually between 1-4 years, incorporating 12-24 monthly workshops and professional support is consistently provided during this time by qualified learning providers and where appropriate, by Accredited Consultants.
Executive summary
Intelligent Automation
History
Process automation dates back to the Industrial Revolution when manufacturing and sales shifted from being done by hand to machines. Organizations are now able to operate consistently and efficiently thanks to business procedures. Process automation lowers the costs and hazards related to using human labor while enabling increases in production volume and quality. In the past, heavy industries such as chemical, power generation, and pharmaceutical have benefited hugely from automating their manufacturing and business functions. This trend has continued in the modern digital age, where businesses employ digital technology and software tools to automate time-consuming and repetitive operations. Business process automation uses technology to perform tasks that human effort would otherwise carry out. It aims to increase efficiency, reduce errors, and streamline operations, freeing up human resources to focus on more strategic and value-added activities. Examples of business processing include automating payroll processing, customer service management, and order processing.
1960s–1970s: The Dawn of Automation
The 1960s and 1970s witnessed the emergence of early process automation systems during the mainframe era. These systems, though primarily used to automate simple repetitive tasks such as payroll processing and inventory management, marked a significant leap forward in improving the efficiency and accuracy of business operations. Despite being custom-built, expensive, and requiring specialized knowledge to operate and maintain, these systems demonstrated the potential of process automation in reducing manual labor and enhancing productivity.
Fact: By the 1970s, IBM’s mainframes were handling payroll processes for major corporations, reducing payroll processing times by over 80%.
1980s: Enterprise Software Revolution
The 1980s marked a significant shift in the evolution of process automation. This era saw the rise of enterprise software and associated processes designed to integrate various business functions like finance, HR, and customer services. These systems provided a more comprehensive approach to automating business processes, allowing organizations to streamline operations across different departments and functions. More importantly, this period marked a transition from custom-built, expensive systems to more standardized, off-the-shelf solutions that could be implemented across multiple industries. This evolution in process automation not only improved the efficiency and accuracy of business operations but also made the technology more accessible and cost-effective, paving the way for a more efficient and streamlined future.
Case Study: SAP introduced one of the first Enterprise Resource Planning (ERP) systems in the 1980s, revolutionizing how businesses managed their resources, finances, and customer data. By integrating various business functions into a single system, SAP reduced the duplication of data and manual effort, which helped companies save millions annually.
1990s: Workflow Automation and Orchestration
In the 1990s, workflows and orchestration in tools and processes became the focus, and organizations could reengineer their business processes to improve efficiency and optimize performance. Orchestration tools allow businesses to automate and manage complex business processes more effectively.
Example: In 1993, GE implemented a workflow automation system that cut administrative processing time by 50% and reduced error rates, driving better customer service and cost savings.
2000s: Rise of Robotic Process Automation (RPA)
Subsequently, in the 2000s, “bots,” or software agents, came into being. These bots could replicate labor-intensive human actions such as data entry, transaction processing, and customer service. These bots often interacted with multiple systems and tools to automate tasks. It gave rise to Robotic Process Automation, which gained widespread adoption due to its ability to automate processes without requiring significant changes to existing IT infrastructure. It allowed businesses to achieve higher levels of efficiency, accuracy, and scalability with relatively low levels of investment.
Fact: The global RPA market grew to $1.4 billion by 2019, with industries such as banking and insurance automating claims processing, data handling, and customer interactions, resulting in cost savings of up to 40%.
Present Day: The Era of Intelligent Automation
Recent times have witnessed a significant evolution in process automation, marked by the integration of artificial intelligence (AI) and machine learning (ML). This convergence has given rise to Intelligent Automation, also known as Intelligent Business Process Automation (IBPA). Unlike traditional process automation, which is rule-driven and implements business logic and decision actions as rules in various tools, Intelligent Automation systems can learn from data, make decisions, and adapt to changing conditions. This shift has opened up new possibilities for automating complex, decision-driven processes and has been a driving force behind digital transformation initiatives in many organizations.
Case Study: UiPath, a leader in RPA and intelligent automation, helped Zurich Insurance automate their claims handling process, reducing handling time by 40% and improving customer satisfaction.
Today, Intelligent Automation stands as a beacon of digital transformation, reshaping the landscape of business operations and competition in the modern world. Its transformative power inspires optimism for the future, promising a new era of efficiency and innovation. This technology is not just a buzzword but a transformative force that is reshaping industries across the board, from finance and healthcare to manufacturing and retail.
Current Position
Today, Intelligent Automation is not just a buzzword but a transformative force reshaping industries across the board. From finance and healthcare to manufacturing and retail, Intelligent Automation is combining the power of Robotic Process Automation (RPA), AI, ML, Natural Language Processing (NLP), and other advanced technologies to automate complex, cognitive tasks that were once the exclusive domain of human workers. This shift is not just about automating repetitive tasks; it’s about enhancing decision-making, improving customer experiences, and driving business innovation.
As shown below, several key technologies are driving the adoption of Intelligent Automation.
Robotic Process Automation (RPA)
RPA is a foundational technology within IA, automating structured, rule-based tasks such as data extraction, transaction processing, and report generation. It is especially effective for automating high-volume tasks with low complexity, freeing up human workers for more strategic activities.
Fact: McKinsey estimates that RPA can automate up to 45% of workplace activities, resulting in increased productivity and significant cost reductions in operations.
Intelligent Document Processing (IDP)
Intelligent Document Processing (IDP) automates the extraction and organization of data from paper-based documents—such as claim forms, legal contracts, and healthcare records—integrating them into business processes. This technology is aligned with RPA but focuses on digitizing and processing documents to reduce manual labor and improve efficiency.
Example: In the insurance industry, IDP has revolutionized the way health insurers process claims. By using optical character recognition (OCR) and AI, companies can reduce the time spent on claims processing by over 50%, resulting in faster payouts and fewer errors.
Natural Language Processing (NLP)
NLP enables machines to understand and respond to human language, powering applications such as virtual assistants, chatbots, and automated content generation. In customer service management, NLP automates query responses, streamlining interactions and enhancing user experiences.
Case Study: Zendesk, a leader in customer service software, uses NLP to understand and prioritize customer inquiries, resulting in 25% faster response times and improved customer satisfaction.
Artificial Intelligence (AI) & Machine Learning (ML)
AI and ML extend automation beyond rule-based processes by enabling systems to analyze data, recognize patterns, and make predictions. For example, AI chatbots can handle customer inquiries accurately, while ML algorithms can optimize supply chain operations by predicting demand. In healthcare, ML assists in diagnosing diseases based on patient data, while in finance, it can predict stock prices.
Example: Amazon uses ML to optimize its supply chain operations, resulting in 15% lower inventory levels and reduced delivery times, improving customer satisfaction.
Deep Learning & Generative AI
One of the most active areas of development in IA is deep learning, particularly generative AI and large language models (LLMs). These models leverage vast amounts of data to generate new content, providing value in industries like media, healthcare, and legal services. LLMs, such as ChatGPT, are transforming how businesses automate content generation and decision-making.
Fact: OpenAI’s GPT-3 has been widely adopted in business environments for tasks like content creation, virtual assistants, and customer engagement, resulting in faster turnaround times and more personalized experiences.
AIOps & Big Data Analytics
AIOps (Artificial Intelligence for IT Operations) and Big Data Analytics are critical in uncovering hidden patterns and correlations in large datasets that would be impossible to detect manually. By providing businesses with these insights, decision-makers can make data-driven, strategic decisions.
Example: Netflix uses Big Data Analytics to optimize its recommendation engine, driving 80% of viewer activity on its platform.
Implementing Intelligent Automation: A Strategic Framework
Successful IA implementation requires more than just advanced technology. A structured framework and robust operating model are essential to guide the deployment, management, and continuous improvement of IA solutions.
• Reference Architecture: This provides a structured approach for planning, deploying, and maintaining IA solutions across the enterprise. It organizes IA into critical functional areas, such as Omnichannel experiences, Core AI automation, and Human Assist capabilities.
• Lean-Agile Methodology: Combining Lean thinking with Agile methodologies, this hybrid approach maximizes efficiency, eliminates waste, and promotes continuous improvement.
Fact: According to Deloitte, companies implementing Lean-Agile in automation projects see a 20-30% increase in delivery speed and 15-20% improvement in cost efficiency.
• Center of Excellence (CoE): A CoE includes architects, data scientists, RPA developers, and change management experts who collaboratively design, manage, and govern IA solutions. The CoE ensures compliance with regulatory requirements and ethical standards while promoting the adoption of IA across the organization.
Example: Coca-Cola established a CoE for RPA, achieving 60% cost reductions in finance processes through automation.
Future Outlook
Addressing Key Challenges with Intelligent Automation
The adoption of Intelligent Automation (IA) is essential for organizations looking to remain competitive in today’s digital landscape. However, several challenges can arise when implementing IA. Here are just some of the challenges our program will address:
1. Difficulty in Identifying the Right Automation Opportunities
• Many organizations struggle to identify which processes should be automated, often focusing on tasks that yield limited value.
• How the Program Helps: The Intelligent Automation program provides a structured framework for process discovery, using tools like process mining and value stream mapping to help participants identify high-impact automation opportunities, ensuring the focus is on the areas that drive the most value.
2. Lack of Alignment with Strategic Goals
• A major issue for many organizations is the failure to align automation efforts with broader strategic objectives, resulting in disjointed and ineffective automation initiatives.
• How the Program Helps: By integrating enterprise architecture and aligning automation efforts with organizational strategy, the IA program ensures that automation initiatives deliver measurable value and support overall business goals.
3. Limited Technical Expertise and Resources
• Non-technical teams often face challenges when deploying and scaling IA solutions, leading to delays and over-reliance on IT departments.
• How the Program Helps: The IA program introduces low-code/no-code platforms, enabling business users to independently design and implement automation solutions. This reduces reliance on IT resources and accelerates deployment timelines.
4. Resistance to Organizational Change
• Implementing IA frequently encounters resistance from employees due to concerns over job displacement or difficulties adapting to new workflows.
• How the Program Helps: The IA program incorporates comprehensive stakeholder engagement and change management strategies. It ensures that all key players are involved, provides upskilling and training programs, and helps build a business case for IA to ease the transition and address concerns about job displacement.
5. Data Security, Privacy, and Compliance Concerns
• With the increased use of IA, organizations face growing concerns about data security, privacy, and maintaining compliance with regulatory requirements.
• How the Program Helps: The IA program places a strong emphasis on security, privacy, and ethics, equipping participants with the tools to implement robust governance frameworks that ensure compliance and protect against cyber threats.
By enrolling in the Intelligent Automation program, you’ll gain the tools, knowledge, and support needed to overcome today’s challenges and lead your organization into a more efficient, agile, and innovative future. Join us and take the first step toward unlocking the full potential of automation in your business.
Curriculum
Process Planning – Part 1- Year 1
- Part 1 Month 1 Roadmap for Success
- Part 1 Month 2 Governance & Organization
- Part 1 Month 3 Business case
- Part 1 Month 4 Enterprise Architecture
- Part 1 Month 5 Data Management
- Part 1 Month 6 Lean-Agile Delivery
- Part 1 Month 7 Technology Landscape
- Part 1 Month 8 Insight Discovery
- Part 1 Month 9 Innovation Adoption
- Part 1 Month 10 Horizontal Processes
- Part 1 Month 11 Vertical Use cases
- Part 1 Month 12 Security & Ethics
Process Development – Part 2- Year 2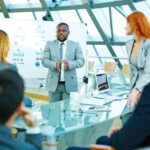
- Part 2 Month 1 Lean-Agile Process
- Part 2 Month 2 CoE & Operating Model
- Part 2 Month 3 Customer Development
- Part 2 Month 4 Discovery & Process mining
- Part 2 Month 5 Process Improvement
- Part 2 Month 6 Benefit Hypotheses
- Part 2 Month 7 Technology selection
- Part 2 Month 8 Data architecture
- Part 2 Month 9 Solution architecture
- Part 2 Month 10 Agile delivery
- Part 2 Month 11 Adoption/Communications
- Part 2 Month 12 Continuous improvement
Program Objectives
The following list represents the Key Program Objectives (KPO) for the Appleton Greene Intelligent Automation corporate training program.
Process Planning – Part 1- Year 1
- Part 1 Month 1 Roadmap for Success – The first step in planning process is to map out the transformation journey in the form of a roadmap. It acts as a blueprint for ensuring that the outcome meets the desired quality, cost, and time constraints. The process kicks off with conceptualization, where the strategy and vision of the Intelligent Automation are laid out. This stage is pivotal as it sets the objective, scope, and requirements of the project, laying the groundwork for subsequent planning. The next crucial step is the readiness assessment of the organisation’s current capabilities and the maturity of its automation practices. It takes into account factors like available resources, existing processes, systems and data quality. The next step in the roadmap delves into a cross-functional center of excellence to lead and support the development of Intelligent Automation. This specialist team consists of members from IT, operations, finance and business units within the organization to drive the development effort. The journey then moves into detailed discovery and analysis of existing business processes to identify use cases with high potential for automation and strong business impact. Stakeholders are engaged to prioritize development activities and to ensure alignment with business objectives. Once the use cases have been identified, an operating model is defined to provide a framework for operational activities as well as associated roles and responsibilities. Organizational change management is an important part of initial planning to prepare the organization for the adoption of automation. It includes communication strategies to educate employees about the benefits of IA and training programs to upskill or reskill the workforce and help with the transition. The next stage is the development of the technical capabilities that enable the automation solutions. It includes selection of appropriate tools and platforms as well as ensuring integration with existing systems. Once the planning stages are completed, the attention turns to execution, to bring the plan to life. The development and delivery of IA solutions follows a Lean-Agile process. Benefit hypotheses are validated and iteratively refined into solutions that add value, reducing waste and increasing the chances of successful outcomes. In this phase, coordination among teams, departments, or stakeholders is vital to maintain smooth execution. After execution follows the monitoring and evaluation phase, where the outcomes are compared against the initial goals. This phase includes defining KPIs and measuring the value and impact of process automations. Lastly, the continuous improvement and sustainability phase are key part of the transformation journey to ensure that the impact of the project lasts long beyond the initial adoption. It’s not just about reaching the end goal, but about constantly refining and enhancing the process.
- Part 1 Month 2 Governance & Organization – “Governance and Organization” refers to the structures, policies, and processes that guide an organization’s decision-making, accountability, and operations. It ensures that the organization operates transparently, ethically, and effectively, aligning with its mission, vision, and goals. Governance typically involves an organization’s oversight and strategic direction, often carried out by a governing body like a board of directors or trustees. This body is responsible for establishing high-level policies, setting long-term objectives, and ensuring the organization complies with legal and regulatory requirements. Governance frameworks define the roles, responsibilities, and powers of those who lead and oversee the organization, ensuring accountability to stakeholders such as shareholders, employees, and the community. Key governance elements include ethical standards, risk management, financial oversight, and strategic planning. At its core, governance emphasizes transparency, accountability, and control. It ensures that leaders make decisions in the organization’s and its stakeholders’ best interest while managing risks and resources responsibly. Organization, conversely, refers to the structure and arrangement of people, roles, and resources within the entity. This includes the formal hierarchy of authority, labor divisions, and communication channels. Organizational design often considers factors like the size of the organization, its goals, and the nature of its work to ensure efficiency and clarity in operations. Organizational structures can range from centralized (with explicit top-down control) to decentralized (with authority spread across different levels). The choice of structure affects how decisions are made, how flexible the organization is, and how efficiently it can respond to changes or challenges. Together, governance and organization create a system that ensures effective leadership, strategic alignment, and operational efficiency while fostering an ethical, compliant, and well-coordinated environment for achieving the organization’s objectives.
- Part 1 Month 3 Business case – A business case is a structured document or presentation that outlines the justification for undertaking a project or initiative. Its primary purpose is to provide decision-makers with the information necessary to determine whether the proposed action is worth pursuing based on potential benefits, costs, risks, and alignment with organizational goals. The business case typically includes financial and non-financial metrics to support its claims. Developing a business case starts with a clear identification of the problem or opportunity. This section not only explains the current situation and the need for change but also underscores the criticality of a well-defined problem. A clear problem definition sets the stage for why the proposed project is not just necessary, but crucial for the organization’s growth and success. Next, the business case outlines the project’s objectives and expected benefits. This section doesn’t just highlight how the initiative will add value to the organization but underscores the importance of these benefits being both measurable and realistic. Whether it’s revenue growth, cost savings, improved processes, or enhanced customer satisfaction, these benefits should be tangible and contribute significantly to the organization’s success. The business case then examines the options for addressing the problem or opportunity. Given the organization’s resources and strategic goals, this includes considering various approaches or solutions and justifying why the recommended one is the best fit. Costs and financial analysis are a significant part of the business case. This section includes a thorough and comprehensive analysis of the project’s expenses, the expected return on investment (ROI), and payback periods. This helps stakeholders understand the financial commitment required and the potential economic returns, instilling confidence in the project’s economic viability. The business case includes a risk assessment that details potential challenges, uncertainties, or obstacles that may impact the project’s success. This section is crucial as it proposes risk mitigation strategies to show how the organization can minimize adverse outcomes, ensuring the project’s success. In conclusion, a well-prepared business case provides a comprehensive argument for proceeding with a project, backed by a thorough analysis of benefits, costs, risks, and strategic alignment.
- Part 1 Month 4 Enterprise Architecture – Enterprise Architecture (EA) is a strategic framework that aligns an organization’s business objectives with its information technology (IT) infrastructure and processes, providing a holistic view that integrates components such as business operations, IT systems, data, and personnel into a cohesive structure. The primary goal of EA is to ensure that technology investments support the organization’s overall mission, improve efficiency, and enable adaptability to change. At its core, EA acts as a blueprint for designing and managing the enterprise’s structure and operations, guiding decision-making by offering a comprehensive understanding of processes, data flows, applications, and technology infrastructure. This alignment guarantees that business strategies are supported by the right technology and that IT investments are optimized for maximum value. EA typically involves multiple layers: Business Architecture focuses on organizational processes and how they support business goals; Information Systems Architecture defines data flow and management; Application Architecture deals with software applications and their interactions; and Technology Architecture concerns the hardware, networks, and platforms that support business operations. A key benefit of EA is standardization, which reduces complexity by unifying processes, systems, and technologies across the organization, lowering costs, reducing risks, and improving overall efficiency. Additionally, EA enables organizations to remain flexible and scalable, allowing them to adapt to new challenges, market conditions, and technologies while ensuring they stay relevant and competitive in a constantly evolving environment. In summary, EA provides a structured approach to aligning technology with business strategy, promoting efficiency, agility, and effective governance.
- Part 1 Month 5 Data Management – Data management is the systematic process of collecting, storing, organizing, maintaining, and utilizing data effectively within an organization. It ensures that data is accurate, secure, accessible, and used efficiently to support decision-making, operations, and strategic goals. As data becomes a critical asset for modern businesses, managing it properly is essential for achieving competitive advantage, compliance, and operational efficiency. Data governance is at the heart of data management, which involves establishing policies, procedures, and standards to ensure data quality, integrity, and security. This includes setting rules for how data is created, classified, stored, shared, and deleted to maintain consistent and trustworthy data across the organization. Governance also addresses compliance with legal and regulatory requirements, such as data privacy laws (e.g., GDPR or CCPA). Data quality management is another crucial component that ensures that data is accurate, complete, and reliable. Poor data quality can lead to incorrect insights, poor decision-making, and operational inefficiencies. Regular data cleansing, validation, and verification processes help maintain high-quality standards. Data storage and security are equally crucial in data management. Organizations need to choose the proper data storage solutions, whether on-premises, in the cloud, or hybrid, to meet their performance, scalability, and cost-effectiveness needs. Data security involves protecting sensitive information from unauthorized access, breaches, and other risks through encryption, access controls, and monitoring. Lastly, data access and analytics play a critical role in making data useful. Effective data management ensures that relevant data is easily accessible to authorized personnel and can be analyzed using tools such as business intelligence platforms, enabling actionable insights. In conclusion, data management is essential for optimizing an organization’s data value, improving decision-making, ensuring compliance, and maintaining data security, accuracy, and accessibility.
- Part 1 Month 6 Lean-Agile Delivery – Lean-Agile delivery combines principles from Lean Manufacturing and Agile methodologies to create an efficient, customer-focused approach to delivering projects and products, widely used in software development and various industries to improve speed, flexibility, and value creation. Lean focuses on eliminating waste, optimizing processes, and maximizing value with minimal resources, originating from Toyota’s production system in manufacturing. Its principles target the removal of inefficiencies in time, effort, and materials to reduce delays and enhance product quality, ensuring that every action adds value to the customer. Agile, on the other hand, is a flexible project management framework that promotes iterative development, customer collaboration, and rapid adaptation to changes. Instead of waiting for a final product after long development cycles, Agile delivers small, usable increments through regular intervals or “sprints,” allowing teams to adapt based on feedback and evolving requirements to ensure alignment with customer needs. When combined, Lean and Agile principles offer a powerful method for delivering value quickly, efficiently, and adaptively. In Lean-Agile delivery, teams are empowered to collaborate, continuously improve, and respond flexibly to changes, all while keeping the customer at the center of the process. Core practices include continuous improvement (Kaizen), where teams regularly evaluate and optimize workflows; value stream mapping, which identifies steps in the delivery process where waste can be eliminated; a strong customer focus that ensures the product meets user needs; and collaborative cross-functional teams that involve stakeholders to maintain aligned goals. In summary, Lean-Agile delivery blends the efficiency of Lean and the adaptability of Agile, enabling organizations to deliver high-quality products faster while remaining responsive to changing demands.
- Part 1 Month 7 Technology Landscape – The technology landscape refers to the broad and dynamic ecosystem of technologies, platforms, tools, and trends that shape industries, businesses, and everyday life. It encompasses many emerging and established technologies, from cloud computing and artificial intelligence (AI) to blockchain, the Internet of Things (IoT), and cybersecurity. This landscape’s constantly evolving nature presents opportunities and challenges for businesses seeking to innovate, stay competitive, and meet customer demands. Cloud computing is at the forefront of the technology landscape, which has transformed how businesses store data, run applications, and scale operations. Platforms like Amazon Web Services (AWS), Microsoft Azure, and Google Cloud offer scalable and flexible computing resources, enabling companies to move away from traditional on-premises infrastructure. The cloud also supports the rise of **Software as a Service (SaaS)** and **Infrastructure as a Service (IaaS)**, providing businesses with on-demand solutions that are cost-effective and efficient. Artificial Intelligence (AI) and machine learning (ML) are other key elements shaping the technology landscape. AI-driven tools are now integral in automating processes, analyzing large datasets, and providing predictive insights across healthcare, finance, and marketing industries. AI’s advancements in natural language processing, computer vision, and robotics are pushing the boundaries of what technology can achieve. The Internet of Things (IoT) is another significant trend, with billions of devices connected globally. These devices exchange data and enable smarter decision-making in areas like home automation, healthcare, and manufacturing. IoT devices gather real-time data that can be analyzed to improve efficiency, safety, and customer experience. Additionally, blockchain technology is revolutionizing industries by providing secure, decentralized systems for transactions, supply chain management, and digital identity verification. While still developing, blockchain can potentially disrupt traditional business models in finance and beyond. Lastly, cybersecurity remains a critical concern, as the rise in digital connectivity increases the risk of data breaches and cyberattacks. Companies must continually invest in security measures to protect their data and maintain customer trust. In summary, the technology landscape is dynamic and interconnected, driven by innovation in cloud computing, AI, IoT, and cybersecurity. Understanding these trends is essential for navigating the opportunities and challenges of modern business.
- Part 1 Month 8 Insight Discovery – Discovery in a business or project context refers to the initial phase of exploring, understanding, and defining the problem space before starting development or execution. It is a critical step in ensuring that the right problem is being solved and that the solution will align with user needs, business goals, and technical feasibility. The discovery phase typically involves a combination of research, collaboration, and validation. The primary goal of this phase is to gather insights that will inform the project’s direction, including understanding the target audience or users, the market landscape, business objectives, and any potential technical constraints. In software development, discovery helps teams identify user pain points, define precise requirements, and prioritize features before moving into the design and development stages. Key activities during discovery often include stakeholder interviews and workshops to engage key stakeholders and understand the business goals, project scope, and success metrics. User research techniques such as surveys, interviews, and persona creation are used to deeply understand user behaviors, needs, and challenges. Additionally, market research is conducted to analyze competitors, industry trends, and existing solutions, helping identify opportunities and differentiators. Technical exploration assesses existing systems, platforms, and tools to identify technical limitations or opportunities. Prototyping and testing are also integral, where low-fidelity prototypes are created to test concepts and gather feedback before committing to full-scale development. The outcome of the discovery phase is typically a set of validated requirements, clear project goals, and an understanding of scope and risks. Deliverables often include user personas, journey maps, wireframes, and a prioritized roadmap. By conducting thorough discovery, teams reduce the likelihood of misalignment, scope creep, or wasted resources, ensuring that the solution addresses the right problem and provides real value to both users and the business, setting a strong foundation for successful project execution.
- Part 1 Month 9 Innovation Adoption – Adoption refers to how individuals, organizations, or markets accept and integrate new ideas, products, technologies, or practices into their daily lives or operations. It plays a critical role in determining the success of innovations, as even the most advanced or beneficial offerings only deliver value when they are effectively used and incorporated. In business and technology, adoption often follows the technology adoption lifecycle, which categorizes users into five groups: innovators, early adopters, early majority, late majority, and laggards. Innovators and early adopters are typically more willing to try new technologies and take on higher risks, whereas the early and late majority prefer to wait until a solution has proven its value and reliability. Laggards, on the other hand, are often the last to adopt, driven by skepticism or a strong preference for established methods. Several key factors influence adoption: perceived value and ease of use, as people are more inclined to adopt technologies that are user-friendly and provide clear benefits, such as increased efficiency or convenience. Cost and accessibility also play a crucial role, as expensive or hard-to-access solutions can deter potential adopters. Social influence and network effects can significantly impact adoption, as endorsements or success stories from peers, influencers, or market leaders build trust and credibility around the new product. Additionally, providing adequate training and support is essential for ensuring users feel confident with a new solution, especially if it involves complex systems or processes. In summary, adoption is about ensuring that innovations are embraced and utilized by their target audiences, and success depends on factors such as perceived value, ease of use, communication, and adequate support throughout the transition. Without effective adoption, even the most innovative solutions may fail to realize their full potential.
- Part 1 Month 10 Horizontal Processes – Horizontal processes refer to cross-functional workflows that span multiple departments or units within an organization, cutting across traditional silos to optimize the end-to-end flow of work. Unlike vertical processes, confined to specific functions like finance, marketing, or HR, horizontal processes connect various functions to deliver value more efficiently and holistically. These processes are critical for ensuring smooth operations and enhancing collaboration across different areas of the organization. Horizontal processes typically focus on delivering outcomes that require input from several departments. For example, a standard horizontal process, order-to-cash, involves coordination between sales, finance, customer service, and shipping teams. Similarly, product development may span marketing, research and development, design, and manufacturing departments. These processes ensure that the organization operates as a cohesive unit rather than in isolated functional silos. The main benefit of focusing on horizontal processes is improved efficiency and collaboration. By breaking down barriers between departments, organizations can reduce redundancy, eliminate bottlenecks, and streamline workflows. This leads to faster decision-making, enhanced innovation, and a more seamless experience for customers or stakeholders. Horizontal processes help align the organization’s efforts with overall business goals, promoting a culture of shared responsibility and teamwork. Implementing horizontal processes often requires process mapping to visualize the flow of work across different functions, identifying inefficiencies and areas for improvement. Technology plays a key role in enabling horizontal processes, particularly with Enterprise Resource Planning (ERP) systems and other integrated software platforms that allow different departments to collaborate in real time and share data. In conclusion, horizontal processes are essential for creating a more connected, efficient, and customer-focused organization. By bridging functional gaps and fostering collaboration across departments, they ensure that the organization works together to deliver high-quality outcomes while reducing costs and improving overall performance.
- Part 1 Month 11 Vertical Use cases – Vertical use cases refer to applications or solutions designed to address a particular industry or sector’s specific needs, challenges, and opportunities, also known as a “vertical market.” These use cases focus on tailored solutions catering to industries’ unique requirements like healthcare, finance, manufacturing, retail, or telecommunications. Vertical use cases are essential in ensuring that technology solutions are relevant and provide value to industry-specific problems. In contrast to horizontal use cases, which apply broadly across multiple industries (e.g., payroll management, CRM systems), vertical use cases are specialized. For example, in healthcare, vertical use cases might include solutions for electronic health records (EHR) systems, telemedicine platforms, or patient management tools, all designed to meet healthcare providers’ regulatory and operational needs. In financial services, vertical use cases could involve fraud detection systems, compliance with financial regulations, or algorithmic trading platforms. Developing vertical use cases requires deeply understanding of industry-specific workflows, regulations, and customer expectations. By tailoring solutions to these needs, businesses can address pain points more effectively and help organizations achieve their goals more efficiently. For instance, smart manufacturing solutions like predictive maintenance systems or automated quality control tools in manufacturing plants are directly aligned with the operational needs of that industry. One advantage of focusing on vertical use cases is the ability to offer niche expertise and tailored value. Vertical solutions providers can position themselves as industry experts, giving them a competitive advantage in the market. Businesses within these industries benefit from solutions that are more precise, compliant with sector regulations, and aligned with their operational processes. In summary, vertical use cases are industry-specific applications that deliver tailored solutions to meet the particular needs of sectors like healthcare, finance, manufacturing, and more. Organizations can better serve industry demands, provide niche expertise, and drive efficiency in specialized areas by focusing on vertical use cases.
- Part 1 Month 12 Security & Ethics – Security and ethics are closely intertwined concepts critical in modern business and technology environments. As organizations increasingly rely on digital systems and manage vast amounts of sensitive data, ensuring security while upholding ethical standards has become a top priority. Security protects information, systems, and networks from unauthorized access, attacks, or damage. This includes safeguarding sensitive data like personal information, financial records, and intellectual property. Adequate security involves a combination of technical measures (such as encryption, firewalls, and intrusion detection systems) and organizational practices (such as policies, employee training, and incident response plans). Inadequate security can lead to data breaches, financial losses, reputational damage, and legal repercussions. In parallel, ethics in technology and business addresses the moral principles that govern technology’s use, management, and impact on individuals, society, and the environment—ethical considerations in security focus on issues like data privacy, user consent, and transparency. For example, companies must collect and use customer data responsibly, with explicit consent, and by legal frameworks like the GDPR (General Data Protection Regulation) or CCPA (California Consumer Privacy Act). Ethical challenges also arise around bias in algorithms, the use of AI in decision-making, and the impacts of surveillance technologies. Organizations are increasingly held accountable for securing their systems and ensuring that their practices do not harm individuals or society. For instance, companies need to avoid using surveillance or data analytics in ways that invade privacy or discriminate against certain groups. In summary, security and ethics are essential in today’s digital age. While security focuses on protecting systems and data, ethics ensures that these protections are implemented in ways that respect privacy, fairness, and societal values. Together, they help build trust, foster responsible innovation, and safeguard individuals and organizations in a rapidly evolving technological landscape.
Process Development – Part 2- Year 2
- Part 2 Month 1 Lean-Agile Process – The Lean-Agile Process is a hybrid framework that combines Lean Manufacturing principles with Agile Software Development to create a streamlined, flexible, and customer-centric approach to managing projects and processes. Its core philosophy centers on eliminating waste, promoting continuous improvement, and delivering value rapidly to customers. Lean principles, originating from Toyota’s production system, focus on identifying and eliminating waste—anything that does not directly add value to the customer—while improving workflow and optimizing resource utilization. Key Lean tools like Kanban are used to visualize work and enhance task flow, while Kaizen encourages continuous incremental improvements. Agile, initially developed for software projects, is a flexible, iterative methodology now applied across various industries. It promotes the delivery of small, functional increments in short cycles, or sprints, allowing for continuous feedback and the ability to adapt to changing customer needs. The integration of Lean and Agile combines the strengths of both approaches, resulting in a process that optimizes delivery speed while maintaining high quality. Agile’s iterative development complements Lean’s focus on reducing waste, and together they encourage cross-functional collaboration, faster decision-making, and alignment with customer value streams. The benefits of the Lean-Agile process are numerous: faster time-to-market due to incremental releases, improved quality through continuous testing and feedback, and a customer-centric approach where regular feedback ensures the product evolves with user needs. Furthermore, the process’s inherent flexibility allows teams to quickly adapt to changes in requirements or market conditions. In summary, the Lean-Agile process fosters a culture of continuous improvement, adaptability, and efficiency, ultimately leading to high-quality products that are delivered faster and provide greater customer satisfaction. For those interested in learning more about the Lean-Agile Process, further reading and resources are available to explore in depth.
- Part 2 Month 2 CoE & Operating Model – Centers of Excellence (CoE) and Operating Models (OpModel) are organizational structures and frameworks designed to enhance operational efficiency, foster innovation, and support the delivery of strategic goals. A Center of Excellence (CoE) is a centralized team or department that focuses on specialized expertise, best practices, and leadership in a particular area such as technology, process optimization, or innovation. CoEs are designed to break down silos, encourage collaboration, and ensure that knowledge and skills are effectively shared across the organization. The primary objectives of a CoE include standardization, where consistent processes and methodologies are established to improve efficiency and reduce errors; knowledge sharing, creating a central hub of expertise to disseminate best practices and innovations; continuous improvement, where the CoE leads initiatives that promote ongoing development and technological advancements; and support, offering specialized skills and resources to business units that may not have them, thereby enhancing project execution and performance. CoEs act as catalysts for organizational transformation, aligning with the company’s long-term strategic goals and driving initiatives that improve overall business outcomes. On the other hand, an Operating Model (OpModel) defines how an organization is structured to deliver value to customers and achieve strategic objectives. It outlines the organization and alignment of resources—people, processes, and technology—enabling efficient operations and growth. The key components of an Operating Model include governance, which provides the decision-making structures, roles, and responsibilities guiding operations; processes that ensure consistent and high-quality delivery of products and services; technology, which includes the systems and tools supporting operations for scalability and automation; and people and culture, where the workforce’s skills, competencies, and the organizational culture directly impact performance and adaptability. Together, CoEs enhance specific capabilities while the Operating Model provides the overall framework to ensure those capabilities are delivered efficiently and effectively across the organization.
- Part 2 Month 3 Customer Development – Customer Development is a systematic process designed to discover, validate, and grow a customer base for a product or service, originating from Steve Blank’s work and forming a key part of the Lean Startup methodology. The approach focuses on understanding customer needs before heavily investing in product development, shifting the emphasis from building a product to validating whether there is a genuine customer demand for it. The process is divided into four critical stages. The first stage, Customer Discovery, involves identifying the target market and potential problems or needs the product aims to address. This phase is centered around testing assumptions and learning from customer feedback through interviews, surveys, and market research to validate or invalidate hypotheses. In the second stage, Customer Validation, the product concept or prototype is introduced to early adopters to determine if there is sufficient demand and whether customers are willing to pay for the solution. Achieving product-market fit at this stage is crucial for ensuring that the product meets the needs of a well-defined market segment. The third stage, Customer Creation, focuses on scaling once the product has been validated. This involves building demand through marketing strategies, sales efforts, and customer acquisition plans, with the goal of expanding the customer base and gaining significant market traction. Finally, in the Company Building stage, the organization transitions from learning and development to growth and execution. With a validated product and a growing customer base, the company’s focus shifts to structuring the business and assembling a team to support long-term success. The importance of Customer Development lies in its ability to minimize the risk of developing products that fail to meet customer needs. By emphasizing early and consistent interaction with customers, companies can pivot or iterate based on genuine feedback, aligning the product with market demands and significantly improving the chances of success.
- Part 2 Month 4 Discovery & Process mining – Discovery and Process Mining are critical tools for understanding and optimizing business processes, enabling organizations to achieve operational efficiency and uncover hidden insights within their workflows. In the context of business processes, discovery refers to the activity of uncovering and mapping out how a process operates in reality. This involves gathering data from various stakeholders, analyzing workflows, and documenting each step of the process. Discovery is essential for understanding the current state of business processes and identifying inefficiencies, bottlenecks, or areas where improvements are needed. There are several approaches to process discovery. Manual Process Discovery typically involves interviews, workshops, and observations to document existing processes, but this method is time-consuming and prone to human error or misinterpretation. On the other hand, Automated Process Discovery leverages tools like process mining to automate the discovery of workflows based on data, reducing manual effort and providing a more objective view of how processes function. Process Mining, a data-driven technique, goes a step further by analyzing business processes through event logs generated by information systems. Unlike traditional discovery methods that rely on subjective input from stakeholders, process mining uses real-time data to generate an accurate and objective representation of business processes. Process mining consists of three fundamental types. The first is Discovery, which automatically generates a current process model from event logs without prior knowledge, offering an accurate depiction of how processes are executed. The second type is Conformance Checking, which compares the discovered process model against an ideal or predefined model to identify deviations and areas of non-compliance. Finally, Enhancement improves the discovered process model by analyzing performance-related information, such as bottlenecks or delays. Process mining enhances traditional discovery approaches by using accurate, real-time data to map out processes more precisely. This allows organizations to quickly identify inefficiencies, optimize workflows, and improve overall business performance. By combining discovery and process mining, businesses are empowered to make data-driven decisions that foster continuous improvement in their operations.
- Part 2 Month 5 Process Improvement – Process Improvement is a systematic approach to identifying, analyzing, and enhancing existing business processes to optimize efficiency, reduce waste, and improve outcomes. It focuses on making incremental changes to boost the performance, quality, and speed of organizational workflows. For organizations seeking to remain competitive, agile, and responsive to evolving market demands, process improvement is essential. There are several critical methods of process improvement. Lean, originating from the Toyota Production System, aims to eliminate waste by removing activities that do not add value and streamlining processes to increase customer value while minimizing unnecessary steps, resources, and costs. Six Sigma is a data-driven approach that focuses on reducing process defects and variations. By utilizing statistical analysis, Six Sigma helps organizations identify inefficiencies and defects, aiming for near-perfect processes. The methodology follows the DMAIC (Define, Measure, Analyze, Improve, Control) framework to guide these efforts. Kaizen, a Japanese philosophy, emphasizes continuous improvement through small, incremental changes and encourages employee participation at all levels, fostering a culture of improvement throughout the organization. Business Process Reengineering (BPR), in contrast to incremental approaches, involves radically redesigning processes to achieve significant performance improvements, often used when current processes are too outdated or inefficient for gradual improvements. Total Quality Management (TQM) focuses on achieving long-term success by emphasizing customer satisfaction and involving all employees in process improvement efforts, creating a culture focused on quality. Process improvement plays a crucial role in enhancing operational efficiency, reducing costs, and increasing productivity. By continuously refining processes, organizations can respond more effectively to market changes, improve customer satisfaction, and maintain a competitive edge. It also helps align business processes with organizational goals, fosters innovation, and increases the quality of products or services. In summary, process improvement empowers organizations to achieve operational excellence by streamlining workflows, reducing waste, and ensuring high-quality outcomes.
- Part 2 Month 6 Benefit Hypotheses – Benefit Hypotheses are statements that predict the positive outcomes or value that a new initiative, product, or feature will bring to customers or the business. These hypotheses are a crucial part of product development and innovation processes, helping teams focus on creating solutions that deliver real value, rather than simply building features for the sake of development. A benefit hypothesis is framed as an assumption that can be tested and validated through experimentation or customer feedback. There are several critical aspects to a well-constructed benefit hypothesis. Customer-Centric Focus is key, as the hypothesis should be centered around solving a customer need or pain point, outlining how a particular feature or solution will improve the user experience, solve a problem, or add tangible value to the customer’s life or work. Testability is another essential element, as the hypothesis must be measurable and testable. Teams must define metrics or success criteria that can be used to validate whether the predicted benefit has been achieved. For example, if a hypothesis suggests that adding a new feature will increase user engagement, specific metrics like time spent on the platform or the number of logins will be used to assess its validity. A strong Link to Business Goals is also important, ensuring that the benefit hypothesis aligns with broader organizational objectives such as increasing revenue, enhancing customer satisfaction, or improving market share. This alignment ensures that initiatives contribute to long-term business success while delivering measurable benefits. Iterative Learning plays a critical role in the validation process, particularly in Lean and Agile methodologies, where teams learn from honest customer feedback and data. If a hypothesis is invalidated, teams can pivot or refine their approach to develop solutions that drive actual value. Benefit hypotheses are essential for reducing risk and ensuring that product development efforts are aligned with customer needs and business objectives. By framing initiatives around testable outcomes, organizations can prioritize features that drive meaningful results, minimize wasted resources, and maximize the impact of their solutions.
- Part 2 Month 7 Technology selection – Technology Selection refers to the process of evaluating, choosing, and implementing the most appropriate technology solutions to meet a business’s specific needs and objectives. This critical process enables organizations to remain competitive, improve operational efficiency, and support ongoing innovation. The right technology selection directly impacts business performance, productivity, and customer satisfaction. The technology selection process begins with understanding business requirements, where the first step is to clearly define the business goals, objectives, and specific needs the technology must address. This includes identifying the problem the technology is intended to solve and outlining the expected outcomes. Gathering stakeholder input ensures that the selected solution aligns with both operational needs and the organization’s strategic goals. The next phase is research and evaluation, where available technologies are assessed based on factors such as functionality, scalability, cost, ease of use, and integration capabilities. Vendors, software solutions, or platforms are compared, often using technical specifications, user reviews, and case studies to guide the decision-making process. A critical consideration is the fit for existing infrastructure, ensuring that the chosen technology integrates seamlessly with the company’s current systems, tools, and workflows to minimize disruptions and maximize the return on investment. A thorough cost vs. benefit analysis follows, weighing the potential benefits of the technology against the total cost, including licensing fees, maintenance, training expenses, and long-term scalability. Pilot testing or running proofs of concept (POCs) before full-scale implementation allows organizations to validate how well the technology meets their needs in practice. Technology selection is crucial because selecting the right technology ensures that businesses make informed, strategic decisions that drive efficiency, foster innovation, and enhance customer experiences. Conversely, poor technology selection can lead to wasted resources, inefficiencies, and failure to achieve business objectives. As such, a thoughtful, data-driven approach to technology selection is essential for long-term success.
- Part 2 Month 8 Data architecture – Data Architecture refers to the structured design and organization of data systems, detailing how data is collected, stored, processed, managed, and utilized across an organization. Serving as a blueprint for managing data assets, it plays a critical role in supporting business objectives by ensuring that data is consistently available, secure, and aligned with enterprise goals. The key components of data architecture include data sources, which are the systems and platforms where data originates, such as databases, cloud applications, IoT devices, or external sources. A well-constructed data architecture integrates these sources to ensure a seamless flow of information and easy accessibility. Data storage involves the infrastructure used to store data, including data warehouses, data lakes, relational databases, and cloud storage systems. This part of the architecture defines how different data types—whether structured, unstructured, or real-time—are stored in a way that ensures scalability and performance. Data integration is another vital component, outlining how data from multiple sources is combined and transformed to provide a unified view of information. This often involves processes like ETL (Extract, Transform, Load), data pipelines, and APIs to ensure that data from disparate systems is consolidated for analysis and reporting. Data governance is integral to the architecture, incorporating policies to manage data quality, consistency, security, and privacy. Governance frameworks ensure that data is accurate, regulatory compliant, and only accessible by authorized users, preventing data breaches or misuse. Finally, data access and usage defines how data is accessed, queried, and analyzed throughout the organization. Data architecture must support various user needs, such as real-time analytics, reporting, machine learning, and business intelligence, delivering data in a usable format to stakeholders. A well-designed data architecture enables organizations to unlock the full potential of their data by ensuring efficient management, easy accessibility, and strong security. It supports data-driven decision-making, facilitates business intelligence, and ensures that data is aligned with business goals. Without a solid data architecture, companies risk encountering data silos, poor data quality, and inefficiencies that hinder their ability to leverage data effectively.
- Part 2 Month 9 Solution architecture – Solution Architecture involves designing and defining the structure, components, and interactions of a technology solution that addresses specific business needs or challenges. It acts as a bridge between business requirements and technical execution, ensuring the technical design aligns with an organization’s strategic goals while being feasible and sustainable. The key components of solution architecture begin with understanding the business requirements, which include the organization’s goals, constraints, and priorities. These requirements shape the solution’s design, ensuring it meets business objectives like improving customer experience, reducing costs, or enhancing operational efficiency. System design follows, where the architect creates the overall structure of the system, specifying how components such as databases, user interfaces, applications, and external systems will interact. This includes choosing the architecture style (e.g., microservices, client-server) and selecting the appropriate technology stack to meet the business needs effectively. Integration is a crucial element of solution architecture, as it ensures that the new solution integrates smoothly with the organization’s existing systems and infrastructure. This involves planning for data flows, APIs, middleware, and ensuring compatibility with other applications within the organization’s technology ecosystem. The architect must also consider scalability and performance, ensuring the solution can grow alongside the business and meet performance requirements like speed, reliability, and availability, especially for mission-critical systems. Security and compliance are integral to the architecture, as the solution must include best practices to safeguard against vulnerabilities and meet regulatory standards. This involves defining access controls, encryption, data privacy protocols, and other security measures. Lastly, documentation and communication are essential, as the architect provides detailed documentation to guide developers, engineers, and stakeholders. Clear communication ensures that everyone involved in the project understands the technical requirements and design decisions. Solution architecture is crucial for ensuring that technology solutions are designed to meet business needs cost-effectively, securely, and with scalability in mind. It mitigates risks associated with project execution by providing a clear technical roadmap, ensuring alignment between implementation and business objectives. Without a well-defined solution architecture, projects are more likely to encounter integration issues, inefficiencies, and fail to meet performance or security standards.
- Part 2 Month 10 Agile delivery – Agile Delivery is an approach to project management and product development that emphasizes flexibility, iterative progress, and continuous value delivery to customers. Rooted in the Agile Manifesto, it prioritizes customer collaboration, working software, responsiveness to change, and individuals over rigid processes and documentation. Agile delivery focuses on producing small, incremental improvements through short, focused development cycles, often called sprints or iterations. Iterative development is a critical aspect of Agile, where teams deliver work in repeated cycles, typically lasting 1 to 4 weeks. Each sprint focuses on delivering a usable piece of the product (a working increment), allowing for continuous refinement and adaptation based on feedback and evolving requirements. Agile is inherently customer-centric, emphasizing delivering value to customers early and often. This approach encourages ongoing feedback from stakeholders and end users, ensuring that the product meets their needs and expectations. By involving customers throughout the process, teams can quickly adjust to changing requirements and deliver a product that aligns with user needs. Cross-functional teams are essential in Agile delivery, as they bring together developers, testers, designers, and product owners to collaborate closely on delivering high-quality products. This collaboration helps reduce bottlenecks, streamline workflows, and accelerate the development process. Agile also fosters a culture of continuous improvement, where after each sprint, teams hold retrospective meetings to evaluate what worked well, what didn’t, and how they can improve in the next cycle. This constant learning and adaptation make teams more efficient and effective over time. Transparency and collaboration are other key aspects of Agile, promoted through regular ceremonies like daily stand-ups, sprint planning, and reviews. These meetings keep the team and stakeholders informed about progress, challenges, and any changes, fostering collaboration and trust throughout the development process. Agile delivery is important because it allows organizations to be more adaptive in fast-changing environments. It encourages early and frequent value delivery, reduces risk by continuously testing assumptions, and keeps the customer at the center of the development process. By focusing on flexibility, collaboration, and continuous feedback, Agile delivery leads to higher-quality products and a faster time to market.
- Part 2 Month 11 Adoption/Communications – Adoption and Communications are critical components of successful change management, particularly when introducing new organizational processes, tools, or systems. Adoption focuses on ensuring that new initiatives are embraced, effectively used, and integrated into daily business operations. Successful adoption requires more than just training; it involves a comprehensive strategy to ensure the change is seen as beneficial and sustainable. Early and consistent engagement with users and stakeholders fosters a sense of ownership, increasing the likelihood of adoption. Providing robust training and ongoing support helps users become comfortable with new tools or processes. Measuring adoption through usage metrics, feedback, and surveys allows organizations to track the effectiveness of the change and identify areas for improvement. On the other hand, effective communication is essential for driving awareness, understanding, and engagement. Crafting a compelling change narrative that explains why the change is necessary and how it will benefit users is key to overcoming resistance and building buy-in. A multi-channel communication approach—using emails, meetings, webinars, and intranet updates—ensures stakeholders receive messages in the formats they engage with most. Two-way communication, allowing for dialogue and feedback, maintains trust and keeps users actively involved throughout the transition. In summary, adoption and communication strategies work hand-in-hand to ensure changes are accepted, embraced, and successfully integrated, driving long-term success and improving business outcomes.
- Part 2 Month 12 Continuous improvement – Continuous Improvement is a systematic and ongoing approach to enhancing an organization’s processes, products, and services, focusing on making incremental changes over time to improve efficiency, quality, and customer satisfaction. Its core aim is to foster a culture of constant learning, adaptation, and innovation, which enables organizations to remain competitive and responsive to market changes. One of the key principles of continuous improvement is incremental change, where minor adjustments are made consistently, leading to significant results over time without causing major disruptions. This makes improvements more manageable and sustainable for the organization. Employee involvement is also central, as engaging workers at all levels encourages collaboration and ownership, with frontline employees often best positioned to identify inefficiencies and suggest practical solutions. Another crucial principle is customer focus, where organizations continually review customer feedback and align their processes to meet or exceed expectations, ensuring that they deliver ongoing value. Continuous improvement also relies heavily on data-driven decisions, using metrics and key performance indicators (KPIs) to measure progress and validate the impact of changes. Popular methods that support continuous improvement include Kaizen, which emphasizes small, incremental improvements; Lean, which focuses on reducing waste and optimizing processes; and Six Sigma, which uses data analysis to minimize defects and improve quality. The importance of continuous improvement cannot be overstated, as it helps organizations optimize operations, increase productivity, and reduce costs while maintaining high-quality standards. By fostering a culture of constant growth and learning, businesses can adapt to changes in their environment, stay ahead of the competition, and enhance customer satisfaction, leading to more sustainable long-term business performance.
Methodology
Intelligent Automation
Program Planning
The Intelligent Business Process Automation training program is meticulously designed for business and technology leaders of companies aiming to automate their business processes. It is not just about adopting the latest trends and best practices in AI, but also about ensuring that the program is strategically aligned with your company’s goals. Participants will be guided on a transformative journey, introduced to key concepts, brought on board all key stakeholders, and assured that the program is in sync with the company’s strategic aims.
The training program is structured to help organizations develop a framework for identifying opportunities for automation in existing processes and re-engineering processes in the context of AI-based technical capabilities. The framework takes the Lean-Agile approach to deploying transformational change in a continuous improvement manner while eliminating waste. It ensures that value is being delivered right from the program’s outset and promises a journey of continuous improvement for long-term benefits.
The first 12 modules aim to lay the foundations for successful IBPA implementation.
A maturity model for IA is presented, and participants will be able to determine what level of maturity their company is at, identifying key stakeholders and the organizational impact of such a change. The benefits of IA will be articulated, ensuring a solid business case for the IA program. Enterprise architecture plays a vital role in ensuring standards and compliance and establishing a data management strategy. Participants will be appraised of the latest tools and best practices in IA and examples of commonly used horizontal and vertical processes to flavor IA in practice. Finally, the program will cover security, privacy, and ethics, as IA can impact these.
Program Development
By this stage, participants will have a good knowledge of Intelligent Automation concepts, how they can be applied to various business processes, and how they impact multiple aspects of an organization. It’s time to devise a process framework that includes the reference functional architecture and a deployment methodology. The framework also consists of an operating model that helps optimize the development and operation of IA solutions. It defines the plan, builds, and operates functions, as well as the influencers and users of the automated solutions. An IA Center of Excellence defines the governance structure and helps manage the solution. It also provides skills and technology to design, develop, and manage automation. It guides technology selection, resource management, and benefits realization at scale.
The modules in this phase are focused on developing a robust Lean-Agile deployment methodology for IA solutions. This approach is designed to ensure not only operational efficiency but also the flexibility to adapt quickly to evolving business needs. By breaking down complex automation projects into smaller, manageable tasks and using iterative development cycles, this methodology promotes continuous feedback and improvement, allowing for adjustments as requirements shift.
The emphasis on Lean-Agile methodology reassures participants that the program is built to deliver tangible results while remaining agile enough to respond to unforeseen challenges or new opportunities. Whether your business is experiencing rapid growth or navigating industry changes, the Lean-Agile approach ensures that automation initiatives remain aligned with strategic goals and can be refined over time to meet emerging demands.
The IA process starts with process discovery using tools and procedures for mining process data and documentation to understand the current state of processes. Value stream maps identify and optimize the flow of value to the consumer. There may also be opportunities for process re-engineering or innovation, particularly within AI and other new technologies. This helps create demand in the IA backlog. The CoE then designs the end-to-end process with automation, which the process owners and business sponsors assess. The solution is evaluated by business and enterprise architects, and an epic hypothesis statement is created.
The epic hypothesis is then validated through a prototype in a Digital Twin or a testbed using Low/no-code automation tools. Once validated, the solution is implemented and deployed using Agile release trains and program increments. In addition, appropriate communication and training plans are also deployed.
Program Implementation
At this stage, participants will have developed and documented the IA process. The operating model and CoE will also be reported, and the organization should be ready to deploy IA solutions at scale.
Participants will learn how to mobilize the CoE and other teams to help deploy IA. The CoE’s first job will be to implement the governance framework and the operating model, including the policies, standards, and best practices. A product selection exercise will be conducted to ensure the appropriate products are selected for the technical solution in line with the organization’s strategic aims. A product catalog will be maintained for future reference.
Program Review
The final set of modules is dedicated to continuous improvement and the ongoing adoption of emerging technologies to maximize business value. These modules emphasize a forward-looking approach, ensuring that Intelligent Automation remains a dynamic and integral part of every business process. By fostering a culture of continuous learning and innovation, the program ensures that organizations are not just reacting to technological advancements but proactively incorporating them into their operations.
This focus on continuous improvement guarantees that new ways of working are continually being designed and optimized around Intelligent Automation, allowing businesses to stay competitive in an ever-evolving marketplace. As emerging technologies become available, the program equips participants with the tools and knowledge to seamlessly integrate them, ensuring sustained efficiency and innovation across all functions.
Industries
This service is primarily available to the following industry sectors:
Financial services
History
The automation of financial services began in the mid-20th century with the introduction of primary machines like mechanical calculators and punched card systems. These tools were initially used for routine tasks like bookkeeping, payroll processing, and data tabulation. The 1950s and 1960s saw the introduction of mainframe computers, which revolutionized financial services by enabling the automation of more complex processes. Automated clearinghouses and ATMs allowed for faster payment processing and 24/7 access to banking services. In the 1970s, electronic funds transfer systems further automated money movement between financial institutions. The 1980s and 1990s saw the advent of electronic trading platforms, algorithmic trading, and stock exchange automation. Digital banking emerged, with banks offering online services and credit scoring systems. The late 1990s and early 2000s saw the rise of fintech companies, which further disrupted the financial services industry. High-frequency trading (HFT) emerged as a dominant force in financial markets, relying on sophisticated algorithms for trade execution.
Current position
Automation in financial services is characterized by the widespread adoption of AI, machine learning, blockchain technology, and robotic process automation (RPA). These technologies streamline operations, enhance decision-making, and improve customer experiences. With millennials becoming a significant part of the customer base, banks are now focusing on providing a customer-focused, engaging experience with real-time virtual services and service interactions at a low cost. The future of automation in financial services will likely be shaped by the continued integration of AI and machine learning, the rise of decentralized finance (DeFi), and the increasing use of big data analytics to drive personalized financial products and services. RPA is a foundational technology in intelligent automation, enabling financial institutions to automate repetitive tasks such as data entry, transaction processing, and regulatory reporting. AI and machine learning are also integrated into decision-making processes, leading to more precise and dynamic decision-making. However, the high cost of regulatory compliance and penalties is impacting profitability in banks. Automation is increasingly used to manage compliance with complex regulations and mitigate risk.
Using technology in customer service can significantly improve the efficiency and customer experience in various sectors. For instance, a good customer experience at a contact center can increase business and upselling opportunities. Chatbots can enable self-service and personalization and reduce the workload of customer service agents. Similarly, banks can enhance their operations around loans, ensuring high operational efficiency and compliance adherence. The application of technology, such as AI and RPA, can transform the loan application and document submission processes, allowing for better validation and screening of documents. This can be done through a combination of manual and automated processes.
Bank fraud operations are also crucial, with various sub-functions such as scams, card fraud, non-card fraud, and delayed payments. These can be identified and reported through channels like customers, bank systems, or third parties like audits and whistleblowers. The fraud operations process at a high level involves preparing and collating data from various sources, analyzing it to identify patterns and relationships, and responding to alerts. The maximum potential of improving efficiency and KPIs with technology lies in fraud detection, investigation, and decision-making.
Future outlook
Conversational virtual agents will be used for various innovative applications, including extreme personalization, virtual reality, and touchless transactions. These technologies can detect life events, recommend products and services, and authenticate customers based on biometrics. AI-driven automation will enhance decision-making processes by providing accurate predictive analytics, enabling more personalized financial advice and optimized investment strategies. As customer expectations for instant, 24/7 service grow, financial institutions will deploy these tools to enhance customer satisfaction and loyalty. AI-powered chatbots, virtual assistants, and NLP tools will evolve to handle complex queries, provide financial advice, and execute transactions on behalf of clients. Integrating blockchain and distributed ledger technologies will enable secure, transparent, and efficient transaction processing. As regulatory environments become more complex, intelligent automation will manage compliance and reduce risk. Ethical AI and responsible automation will be crucial, leading to industry-wide standards and regulatory scrutiny. The widespread adoption of intelligent automation will transform the workforce in financial services, requiring upskilling and reskilling workers to adapt to new roles.
Healthcare
History
Automation in healthcare has evolved from the mechanization of laboratory processes to the integration of AI in diagnostics. The earliest forms of automation can be traced back to the late 19th and early 20th centuries, with mechanized tools introduced for surgical procedures and diagnostic tasks. The 1960s and 1970s saw the introduction of computers for administrative tasks and the development of electronic health records (EHRs). In the 1970s and 1980s, computerized tomography (CT) scanners and automated pharmacy systems revolutionized diagnostic imaging and medication dispensing. The 1990s saw the introduction of robotics, such as the da Vinci Surgical System, which improved surgical outcomes and reduced recovery times. Automation has advanced in laboratory diagnostics, radiology, and administrative processes. AI and machine learning are increasingly driving automation in healthcare, enhancing diagnostic accuracy, predicting patient outcomes, and personalizing treatment plans. The COVID-19 pandemic has accelerated the adoption of automation in healthcare, with telemedicine and vaccine distribution systems playing critical roles.
Current position
The healthcare industry is experiencing a significant shift towards intelligent automation, with advanced technologies like artificial intelligence (AI), machine learning (ML), robotic process automation (RPA), and natural language processing (NLP) being integrated into various aspects. AI and machine learning are revolutionizing diagnostics and clinical decision-making by analyzing medical images faster and more accurately. CDSS systems also benefit from AI, which provides real-time data and evidence-based recommendations and improves the quality of care. Robotic surgery systems, like the da Vinci Surgical System, are becoming more prevalent in hospitals, allowing for minimally invasive procedures with higher precision. RPA is being adopted to streamline administrative tasks, such as patient scheduling, billing, claims processing, and data entry. AI-driven tools also improve patient engagement through chatbots and virtual health assistants, which can answer patient queries, schedule appointments, provide medication reminders, and offer health advice. Telemedicine platforms incorporate AI to triage patients, suggest potential diagnoses, and recommend next steps based on symptoms reported online. Automation is also crucial in managing healthcare supply chains, predicting inventory needs, tracking supplies in real time, and managing procurement processes. However, integrating intelligent automation in healthcare faces challenges such as data privacy concerns, significant investments, job displacement, complex and costly integration, and ensuring unbiased and transparent AI and automated systems.
Future outlook
The future of healthcare is set to be transformative, with AI and machine learning playing a critical role in diagnostics, telemedicine, robotics, and rehabilitation. AI systems will analyze complex datasets to predict disease risks and tailor treatment plans, improving patient outcomes and reducing healthcare costs. Telemedicine will see an expansion in AI-powered remote monitoring tools, enabling real-time tracking of patient health metrics. Robotic systems in surgery will become more sophisticated, offering greater autonomy and personalized care. Blockchain technology and intelligent automation will revolutionize data storage, sharing, and security. AI-driven tools will empower patients to manage their health actively, providing personalized advice and monitoring medication adherence. AI and machine learning will accelerate drug discovery and development, reducing time and cost. However, ethical issues and regulatory compliance remain critical.
Utilities
History
Automation in the utilities industry has evolved from mechanical systems to digital and AI-driven solutions. The earliest forms of automation occurred in the late 19th and early 20th centuries, with mechanization in power generation and distribution. The 1950s saw the introduction of Supervisory Control and Data Acquisition (SCADA) systems, which allowed utilities to monitor and control processes across vast networks. The digital revolution of the 1980s and 1990s further transformed the industry, leading to the “smart grid” concept in the electricity sector.
In the 21st century, utilities increasingly embrace advanced automation technologies driven by the Internet of Things (IoT), artificial intelligence (AI), and machine learning. IoT devices monitor infrastructure, predict maintenance needs, and optimize resource flow. AI algorithms forecast energy demand, manage grid stability, and detect leaks in water distribution systems. Automation has expanded into customer-facing operations, with chatbots and automated customer service systems handling routine inquiries and billing.
Automation is crucial for managing the complexities of integrating renewable energy sources into the energy sector’s grid. However, cybersecurity remains a primary concern, and the transition to fully automated systems requires significant investment and workforce adaptation.
Current position
Intelligent automation is transforming the utility industry by integrating advanced technologies like artificial intelligence, machine learning, the Internet of Things (IoT), and robotic process automation. These technologies transform how utilities manage infrastructure, interact with customers, and adapt to evolving energy demands. Smart grids use AI and IoT to monitor electricity flow in real time, enabling better demand forecasting, load balancing, and integration of renewable energy sources. Predictive maintenance is another primary application of intelligent automation, leveraging IoT sensors and AI-driven analytics to monitor asset health and predict potential failures.
Utilities also use AI-powered chatbots and virtual assistants to handle customer inquiries, process payments, and manage service requests. These systems provide 24/7 support, improving customer satisfaction and reducing the operational burden on human staff. Automation also enhances energy efficiency and facilitates demand response programs, enabling real-time data on energy usage and automatic adjustment during peak times.
Integrating renewable energy into the grid is a significant challenge, and automation helps manage the variability of renewable energy sources by optimizing their contribution to the grid in real-time. Automation is also essential for regulatory compliance and data management, as it helps utilities comply with safety, environmental impact, and customer data privacy requirements.
Future outlook
The utilities industry is set to see significant advancements in the next decade, driven by the integration of AI, machine learning, and IoT technologies. Smart grids will evolve, with decentralization of energy systems enabling efficient management of distributed energy resources. Automation will also facilitate greater customer participation in the energy market, optimizing energy flow and dynamic pricing models. AI-powered predictive maintenance will improve resource planning and decision-making, reducing operational costs and improving service reliability. Cybersecurity will be a top priority, with AI-driven threat detection and response systems protecting critical infrastructure. Data management will become more sophisticated, with AI and machine learning processing vast amounts of data generated by IoT devices and smart meters. AI-powered chatbots and virtual assistants will handle complex customer interactions, providing real-time responses and personalized energy management solutions. AI and ML will optimize renewable energy use, meet sustainability goals, and enhance water management. As AI becomes more embedded in utility operations, regulatory frameworks must evolve, focusing on data privacy, cybersecurity, and ethical use.
FMCG Retail
History
Automation in the FMCG retail industry has evolved from mechanization to AI and IoT technologies. The earliest forms of automation focused on mechanizing production processes, such as assembly lines and cash registers. The mid-20th century saw the expansion of automation to more complex systems in manufacturing and distribution. The Universal Product Code (UPC) in the 1970s automated the checkout process, reducing transaction time and improving inventory accuracy. The 1980s and 1990s saw a shift towards computerization, with sophisticated Point of Sale (POS) systems, Enterprise Resource Planning (ERP) systems, and electronic data interchange (EDI) automating communication between retailers and suppliers. The digital revolution in the 21st century transformed how consumers purchased goods and how retailers managed operations. Today, automation in the industry is characterized by integrating advanced robotics, AI, and IoT technologies. Robots are increasingly used in warehouses for picking and packing products, while AI-driven systems manage inventory, forecast demand, and personalize the shopping experience. IoT devices enable real-time monitoring of inventory levels and product conditions, and cashierless stores are being explored.
Current position
Intelligent automation is revolutionizing the Fast-Moving Consumer Goods (FMCG) retail industry by leveraging artificial intelligence, machine learning, robotic process automation, and the Internet of Things. It transforms supply chains, enhances customer experience, and makes data-driven decisions. AI and ML algorithms predict consumer behavior, adjust supply levels, and ensure timely product delivery. Robotics and automated guided vehicles are increasingly used in warehouses, increasing efficiency and reducing human error.
Customers are also utilizing AI-driven chatbots and virtual assistants to provide personalized recommendations and assistance with the purchasing process. Retailers also leverage AI to personalize marketing strategies based on individual consumer data. Self-checkout systems and cashierless stores are also being adopted in physical retail spaces. Digital shelf labels, automated inventory monitoring, and other in-store technologies are also used to optimize operations.
Sustainability is a growing focus in the FMCG industry, and automation is playing a significant role in this transformation. It’s reducing waste and improving the industry’s environmental impact by optimizing packaging, reducing energy consumption in production and distribution, and managing waste more effectively. AI can optimize delivery vehicle routing to minimize fuel consumption, while automated systems in manufacturing can reduce material waste by improving precision and efficiency. These efforts are a positive step towards a more sustainable future.
As intelligent automation becomes more integrated into FMCG retail operations, it transforms the workforce. Companies are investing in reskilling and upskilling programs to prepare their workforce for these changes and ensure they can effectively work alongside automated technologies.
Future outlook
The FMCG retail industry is set for significant growth and transformation due to AI, ML, and IoT advancements. These technologies will enable sophisticated predictive analytics, optimize pricing strategies, and personalize marketing efforts. Autonomous supply chains will expand, with drones and autonomous vehicles becoming standard for last-mile delivery. AI tools will enable hyper-personalized marketing, product recommendations, and shopping experiences tailored to individual preferences. Sustainability will remain a significant focus, with AI playing a crucial role in reducing waste, improving resource efficiency, and supporting circular economy initiatives. The future of FMCG retail will be defined by seamless omnichannel experiences, with AI-driven chatbots, virtual assistants, and augmented reality tools enhancing online shopping and in-store technologies, providing more efficient and personalized physical shopping experiences. However, regulatory and ethical considerations will play a significant role in shaping automation deployment, with companies needing to navigate challenges such as data privacy, algorithmic transparency, and ethical AI use.
Public sector
History
The history of automation in the public sector can be traced through key milestones. It began in the 1950s and 1960s, when governments started using computers to manage large volumes of data. The 1980s and 1990s marked a significant expansion, driven by the widespread adoption of information technology (IT) systems. This period saw the emergence of e-government initiatives, providing citizens with digital access to public services. The early 2000s witnessed a shift towards e-government and digital transformation, with governments leveraging the internet and digital technologies to automate citizens’ and state interactions. This era also saw the increasing use of automation to streamline online tax filing, electronic voting, and digital identification systems. In recent years, the public sector has embraced more advanced forms of automation, including artificial intelligence (AI) and machine learning (ML). AI-powered chatbots handle routine citizen inquiries, while machine learning algorithms help in fraud detection, predictive policing, and healthcare management. Governments are also exploring robotic process automation (RPA) for repetitive tasks. Smart cities are emerging, deploying IoT devices and AI to manage infrastructure, monitor traffic, control energy consumption, and enhance public safety.
Current position
Intelligent automation is not just a concept, but a rapidly evolving technology that is being implemented worldwide in various public sector functions. It includes technologies like artificial intelligence (AI), machine learning (ML), robotic process automation (RPA), and the Internet of Things (IoT). These technologies are enhancing public service delivery by automating routine administrative tasks, allowing employees to focus on more complex and value-added activities. RPA automates processes like claims processing, tax filings, and benefits administration, leading to faster processing times and reduced backlogs. AI-driven chatbots and virtual assistants are also becoming common in government agencies to handle citizen inquiries, provide information, and guide users through online services. Governments also leverage AI and ML to analyze large datasets and make data-driven decisions, improving public health, urban planning, financial management, and smart cities. IoT and AI are also being used to enhance public safety, monitor traffic patterns and energy consumption in public buildings, and ensure compliance with regulations. This transformation is not a distant future, but a current reality that is reshaping the public sector workforce and how public services are delivered.
Future outlook
Intelligent automation in the public sector is expected to transform significantly due to technological advancements, increased demand for efficient services, and the need to address complex societal challenges. AI, machine learning, robotic process automation, and IoT will become integral to public sector operations, enhancing decision-making processes, improving service delivery, and enabling personalized interactions with citizens. AI models will be used to predict crime hotspots, manage traffic flows, and optimize energy consumption in smart cities. The concept of smart cities will expand, with interconnected devices and sensors monitoring traffic, air quality, waste management, and public safety in real time. Integrating 5G networks will further enhance IoT device capabilities, enabling faster and more reliable communication between systems. As AI becomes more prevalent, the public sector will offer more personalized and accessible digital services, allowing employees to focus on more complex and strategic work. However, ethical and regulatory challenges will arise, such as data privacy, algorithmic bias, and transparency of AI decision-making processes. Governments must invest in reskilling and upskilling programs to prepare employees for these changes, ensuring a smooth transition to the future of work in the public sector. The future of intelligent automation in the public sector will involve increased global collaboration and innovation.
Locations
This service is primarily available within the following locations:
London, UK
History
London has been pivotal in the UK’s technological and automation advancements since the Industrial Revolution in the late 18th century. The steam engine, developed by James Watt, revolutionized transportation and industry. London’s industrial powerhouse status led to the expansion of the railway network in the 19th century, accelerating industrial growth. The Second Industrial Revolution introduced electricity, the internal combustion engine, and chemical manufacturing, leading to greater automation in production processes. The Colossus, the first programmable computer, was invented in 1944, laying the groundwork for modern computing. The post-war telecommunications industry also saw the rise of the digital era. By the late 20th century, London became a global center for finance and technology, with the “Big Bang” deregulation leading to the adoption of electronic trading systems. The 1990s and 2000s saw the rise of the internet and mobile technologies, making London a hub for digital startups.
Current Position
London has been a global leader in technological innovation, particularly in fintech, AI, and automation. The UK is recognized as a worldwide leader in AI development, with London-based companies and research institutions playing a key role. Automation has advanced significantly, with AI being integrated into industries like finance and healthcare. The UK government has promoted London as a global technology hub, with initiatives to support AI, automation, and digital transformation. London’s technology sector is critical to the UK’s economy, contributing significantly to GDP and employment. As of 2024, the UK is the top tech ecosystem in Europe and ranks third globally, with a value of $1.1 trillion. London’s diverse tech ecosystem, including 171 unicorns, attracts international investors, including US-based venture capital firms. AI is a strong focus, with over 1,800 active startups. The UK government has launched initiatives like the “Unicorn Kingdom” campaign to attract foreign investment and promote the UK as a global science and technology leader.
Future outlook
The UK’s technology sector, particularly in London, is poised to remain a leading global hub due to continued investment in AI, fintech, green tech, and digital infrastructure. The city is positioning itself as a worldwide leader in AI, with significant government support. London is home to numerous fintech companies transforming financial services through digital banking, blockchain, and payments. The UK also focuses on sustainability and green technology, with startups and established companies investing in clean energy, electric vehicles, and sustainable supply chains. London’s vibrant startup ecosystem, supportive regulatory environment, and top talent attract international investors. The rollout of 5G and digital infrastructure will enable new technologies like IoT and autonomous vehicles to flourish.
Paris, France
History
Paris has a rich history of technology and automation, dating back to the Industrial Revolution. The city’s rapid growth and economic importance led to the adoption of mechanized production in industries like textiles, mining, and metalworking. The Second Industrial Revolution saw further technological advancements, with Paris being a hub for electrical technology and hosting international exhibitions. The early 20th century saw significant developments in the automotive and aviation industries, with Paris playing a central role. The French automobile industry was one of the earliest and most influential in the world, with companies like Renault and Citroën establishing themselves in the city. Post-World War II, France experienced rapid industrial growth, with Paris leading the electronics and telecommunications industries. The digital revolution began in the latter half of the 20th century, with Paris developing its computing systems and the Minitel, an early precursor to the internet.
Current position
Paris has become a global tech hub, with a surge in the growth of software, telecommunications, and digital services industries. The city has established technology parks and innovation hubs, such as the Paris-Saclay cluster, which have fostered tech startups and advanced research. Paris has also embraced automation and AI, with significant investments in these fields. The French government has supported these developments, launching initiatives to position France as an AI and digital technology leader. In 2022, French startups raised a record €13.5 billion, reflecting the resilience of the French tech scene despite challenging economic conditions. The city’s open-door policy, exemplified by the French Tech Visa, has attracted a diverse pool of global talent, including non-EU entrepreneurs, employees, and investors. The French government has proactively supported tech innovation, with President Macron announcing plans to make Paris the “AI capital of Europe.” The future of the technology sector in Paris looks promising, with continued growth in areas like AI, fintech, and cleantech.
Future outlook
France’s technology sector is expected to grow, with Paris being a key hub. The country’s commitment to innovation and sustainability, backed by strong government support and initiatives like the French Tech Visa, is expected to attract domestic and international investment. Paris also positions itself as a leader in AI and green tech, with the government investing significantly in research, development, and education. The green tech sector is also expected to grow, with Paris leading the way in renewable energy and electric vehicles. However, investors are shifting towards profitability and sustainable business models, potentially leading to market consolidation. The French government’s unwavering support for the tech sector is expected to continue, with initiatives like the French Tech Visa and government-backed programs playing a crucial role in maintaining the industry’s momentum.
Geneva, Switzerland
History
Geneva, Switzerland, has a rich history in technology and automation, a testament to the Swiss people’s resilience and adaptability. Despite the challenging mountainous terrain and a lack of natural resources, the Swiss turned to high-quality, specialized industries. The watchmaking industry, particularly in Geneva, emerged as a global leader. The 19th century witnessed significant strides in electrical engineering, with Swiss companies like Brown, Boveri & Cie playing a pivotal role. Switzerland’s neutrality during the World Wars allowed it to focus on technological development. In the early 20th century, Geneva became a center for international telecommunications, hosting the International Telegraph Union. This period saw automation in manufacturing processes and integration into administrative processes.
Current position
The mid-20th century saw Switzerland’s digital revolution, with Geneva playing a significant role as a hub for international organizations. The country’s contributions to computing include the development of the ALGOL programming language at ETH Zurich in the 1950s. Geneva also saw growth in the tech sector, particularly in banking and finance, where automation became increasingly important. In the late 20th and early 21st centuries, Switzerland continued building on its reputation for high-tech innovation. Geneva became a center for research and development in technology due to its proximity to major international organizations like CERN. Switzerland’s technology sector, particularly in cities like Geneva, is flourishing and poised for continued growth. Switzerland is consistently ranked at the top of the Global Innovation Index due to its emphasis on R&D, innovation, and collaboration with international partners. Geneva’s tech scene is supported by strong academic institutions and government initiatives, with startups making significant strides in sustainable cloud computing and alternative proteins.
Future outlook
Switzerland’s tech sector, including Geneva, is expected to thrive due to emerging technologies like AI, blockchain, and advanced manufacturing. Significant investments and international collaborations further solidify the country’s reputation as a hub for biotech innovation. Switzerland’s focus on sustainability, AI, biotech, fintech, and strong government support will ensure its continued growth and innovation. Geneva, with its unique position as an international hub, will continue to attract global talent, investment, and new tech opportunities, further enhancing its global significance.
Amsterdam, Holland
History
Amsterdam’s history of technology and automation reflects the Netherlands’ early adoption of innovative practices, adaptation to industrial and digital revolutions, and a continuous push towards future technologies. The Dutch Golden Age in the 17th century saw technological advancements in shipbuilding, navigation, and water management. The Industrial Revolution brought significant changes, particularly in Amsterdam, with the introduction of steam engines and mechanized production processes. In the 20th century, Amsterdam became a center for the electrical industry, leading innovations in electrical engineering and consumer electronics. The digital revolution in the late 20th century impacted Amsterdam’s technology landscape, making it a key European hub for internet and telecom companies.
Current position
Amsterdam’s tech hub status has grown significantly in the 21st century, with a thriving startup ecosystem in fintech, AI, and creative industries. Strong government policies, world-class research institutions, and a highly educated workforce support the city’s innovation climate. Amsterdam’s strategic location within Europe and well-established infrastructure, including the Amsterdam Internet Exchange, contribute to its growth. The tech sector in the Netherlands, particularly in Amsterdam, is valued at €71 billion. However, the broader Dutch tech sector faces mixed fortunes, with concerns about the sustainability of profound tech growth. The Netherlands has not produced new “unicorns” in the past year, and Dutch startups often struggle to secure follow-on funding. Despite these challenges, the outlook for the Dutch tech sector remains positive, with a growing focus on deep tech and increasing international investor involvement.
Future outlook
Amsterdam is set to remain a leading European tech hub due to its focus on sustainability, green technology, innovative city initiatives, AI, and automation technologies. The city’s tech scene drives innovation in fintech, health, and sustainable technologies. However, the sector faces challenges in attracting and retaining skilled talent, particularly in AI, quantum computing, semiconductors, and biotechnology. The Netherlands focuses on improving education and training, attracting international talent, and addressing the talent gap to maintain growth. The Netherlands is also committed to green technology, focusing on innovative city initiatives and clean energy solutions. Despite a slowdown in venture capital funding, the long-term outlook remains strong, with international investors showing keen interest in the country’s tech companies. And expansion, particularly in high-growth areas like AI, fintech, and biotech.
New York, NY
History
New York City has a rich history of technological advancements, shaping its future and the global technology landscape. In the 19th century, the city emerged as an industrial powerhouse due to its strategic location and access to raw materials—the steam engine’s introduction and the Erie Canal’s expansion in 1825 boosted industrial capacity. In the late 19th century, significant electrical power and communication advancements, such as Thomas Edison’s first central power station, led to rapid growth and technological advancements. The city also became a center for communication technology with the completion of the transatlantic telegraph cable and the development of the telephone. The mid-20th century saw the beginning of the computer age, with IBM playing a significant role in data processing and punch-card machines. The late 20th century saw New York evolve into a central tech hub, with Silicon Alley becoming a hub for internet startups.
Current position
New York City has been a leader in the tech sector, particularly in fintech, health tech, and media technology. The city’s diverse economy, cultural vibrancy, and access to capital have attracted tech giants like Google, Amazon, and Facebook. New York is also at the forefront of automation and AI development, with innovative city initiatives integrating automation into its infrastructure. Academic institutions like Columbia University and NYU are conducting cutting-edge AI, robotics, and machine learning research to ensure the city remains a global leader in technology and automation. The tech sector has shown remarkable resilience and growth, particularly during challenging times like the COVID-19 pandemic. The city is a significant hub for internet-related tech jobs, holding 15.6% of all jobs in the United States. Venture capital investment in tech startups reached $53.1 billion in 2021. Large tech companies like Google have played a critical role in this growth, and initiatives like “Pilot: New York City” aim to address urban challenges by fostering collaboration between government, academia, and the tech industry.
Future outlook
New York City’s tech sector is expected to grow, focusing strongly on fintech, health tech, and AI. The city’s diverse economy, strong educational institutions, and vibrant startup ecosystem make it a leading global tech hub. The city’s diversified tech ecosystem includes deep tech and real estate startups and established areas like software development and e-commerce. World-class universities like Columbia University and NYU provide a steady talent pipeline, while initiatives to expand tech education, such as coding boot camps, are expected to bolster the talent pool. New York’s strong position in attracting venture capital investment, with startups receiving $53.1 billion in 2021, supports startup growth and helps established companies scale operations. Major tech companies like Google, Amazon, and Facebook are also expected to continue investing in the tech sector.
Program Benefits
Management
- Strategic alignment
- Collaborative management
- Increased efficiency
- Cost reduction
- Agile delivery
- Technical framework
- Operational streamlining
- Improved adoption
- Scalable automation
- Innovation acceleration
Operations
- Cost efficiency
- Revenue growth
- Work throughput
- Increased productivity
- Staff morale
- Process quality
- Flexibility scalability
- Enhanced compliance
- Better oversight
- Reduced debt
Customer Service
- Omnichannel service
- Faster response
- Better quality
- Error reduction
- Customer personalization
- Churn reduction
- Enhanced security
- Smart insights
- Customer satisfaction
- Proactive support
Testimonials
SITA
SITA is a prominent provider of IT Services to the air transport sector, serving 2500+ airline customers across 1000+ airports in 200+ countries. They wanted to replace their voice- and email-based customer service operations with an Omnichannel capability with bot automation. I was brought in to lead the design and delivery of the Omnichannel customer support solution.
The solution resulted in a 40% reduction in call volumes and an 80% reduction in emails in the first year of operation. As a by-product of this work, a patent application was submitted by SITA to capitalize on intellectual property developed as part of the solution.
Digital Space
Digital Space provides cloud services to a number of large organizations including NHS and other public sector organizations in the UK public sector. They wanted to offer the latest AI based technologies to their customers. I was hired to lead the design of an Omnichannel Cloud Contact Centre using public cloud services. The design served as a blueprint for new service offering for the company, resulting in increased market share.
Private Equity firm (under NDA)
A private equity firm had acquired a service provider in Switzerland providing document processing services to its financial services customers and wanted to improve its service offerings to include intelligent document processing features. I was engaged to propose an intelligent document processing solution that would automate the processes involved in insurance claims processing, including first notice of loss, loan assessment and settlement. A second use case was the mortgage loan application processes, including assessment, approval and disbursement. A set of AI and SaaS service in the cloud were used to design a flexible solution that would enable the company to improve its current offering and to also acquire new clients.
Financial Services (under NDA)
Following the acquisition and merger between two large financial services organisations, they were suffering from data quality issues in their operational process automation systems. I was brought in to drive a service improvement programme to address the data quality issues. I reviewed the various business processes and system integrations to determine the root cause of the issue and then worked with the stakeholders to put together a remediation plan. A tactical solution was delivered against tight delivery schedule to meed the requirements of external auditors, and at the same time a more permanent solution was recommended.
Utilities Company (under NDA)
A utilities company in the UK wanted to modernize their HR systems and processes. I worked closely with the HR director to lead a transformation programme. I helped automate case management and other HR processes in the HR centre of excellence. I also introduced chatbots and virtual agents (bots) in various automation. During investigations it was discovered that there were issues with the quality of data being used by automation. I then led a separate effort to remediate the data issues and associated integrations.
More detailed achievements, references and testimonials are confidentially available to clients upon request.
Client Telephone Conference (CTC)
If you have any questions or if you would like to arrange a Client Telephone Conference (CTC) to discuss this particular Unique Consulting Service Proposition (UCSP) in more detail, please CLICK HERE.