AI Strategy
The Appleton Greene Corporate Training Program (CTP) for AI Strategy is provided by Mr. Stambaugh Certified Learning Provider (CLP). Program Specifications: Monthly cost USD$2,500.00; Monthly Workshops 6 hours; Monthly Support 4 hours; Program Duration 12 months; Program orders subject to ongoing availability.
Personal Profile
Mr. Stambaugh has decades of experience designing, planning, and implementing complex technology transformations in public and private organizations. He has led enterprise-level programs focused on Information Security (InfoSec), industrial SCADA deployments, telecommunications modernization as well as advanced analytics / artificial intelligence (AI) / machine learning deployment – and managed complex national technology and operational teams at the VP and director level. He has deep experience in the energy, utilities, geospatial, and telecommunications sectors, operating in Canada and the United States. This experience is supported by a master’s-level technical degree and nearly ten years as a science and technology columnist with the Canadian Broadcasting Corporation (CBC) on radio and national television.
He has leveraged this broad background in technology transformation into a successful Artificial Intelligence (AI) implementation practice, assisting organizations with the complex but critical task of creating an AI strategy and then developing and executing their implementation strategy. He is excited to leverage this experience to support other organizations on their AI journey through this program.
To request further information about Mr. Stambaugh through Appleton Greene, please Click Here.
(CLP) Programs
Appleton Greene corporate training programs are all process-driven. They are used as vehicles to implement tangible business processes within clients’ organizations, together with training, support and facilitation during the use of these processes. Corporate training programs are therefore implemented over a sustainable period of time, that is to say, between 1 year (incorporating 12 monthly workshops), and 4 years (incorporating 48 monthly workshops). Your program information guide will specify how long each program takes to complete. Each monthly workshop takes 6 hours to implement and can be undertaken either on the client’s premises, an Appleton Greene serviced office, or online via the internet. This enables clients to implement each part of their business process, before moving onto the next stage of the program and enables employees to plan their study time around their current work commitments. The result is far greater program benefit, over a more sustainable period of time and a significantly improved return on investment.
Appleton Greene uses standard and bespoke corporate training programs as vessels to transfer business process improvement knowledge into the heart of our clients’ organizations. Each individual program focuses upon the implementation of a specific business process, which enables clients to easily quantify their return on investment. There are hundreds of established Appleton Greene corporate training products now available to clients within customer services, e-business, finance, globalization, human resources, information technology, legal, management, marketing and production. It does not matter whether a client’s employees are located within one office, or an unlimited number of international offices, we can still bring them together to learn and implement specific business processes collectively. Our approach to global localization enables us to provide clients with a truly international service with that all important personal touch. Appleton Greene corporate training programs can be provided virtually or locally and they are all unique in that they individually focus upon a specific business function. All (CLP) programs are implemented over a sustainable period of time, usually between 1-4 years, incorporating 12-48 monthly workshops and professional support is consistently provided during this time by qualified learning providers and where appropriate, by Accredited Consultants.
Executive summary
AI Strategy
We are amid a fundamental transformation. Applications based on artificial intelligence technologies are rapidly evolving, with opportunities for enhancing nearly every commercial process and unlocking significant value for those organizations able to integrate it successfully into their business. It is a market that is projected to be worth more than $2 trillion (US) by the end of the decade and will cause massive disruption across labour markets, eliminating but also creating millions of new jobs.
Every business needs to have an artificial intelligence AI strategy. This strategy will vary in size, scope, and complexity based on the needs of the specific organization, of course, but it is critical to understand the capabilities, benefits, and potential risks of AI-based applications – especially as your clients, partners, and employees are already using this technology to some extent.
What is ‘Artificial Intelligence’?
There are several definitions of Artificial Intelligence (AI), but the common thread is an attempt to create software applications that can approximate (and potentially surpass) human abilities in creative reasoning and problem-solving. The critical difference between AI and other computer programs is their ability to use their past ‘experience’ through training to effectively solve problems that they have not been pre-programmed for – unlike traditional software where a human must provide clear instructions for every eventually the program may face, or an error will be returned.
This ‘general AI’ that can fully mimic humans’ wide-ranging logic and reasoning capabilities in a single program currently does not exist. When the media and companies talk about AI today, they are referring to applications built to fill a specific need, such as ‘chatbot’ to engage and answer questions for humans, image analysis designed to identify medical issues from x-ray or MRI scans, or programs designed to estimate the probability of a maintenance failure based on multiple sensor inputs. That said, as software such as ChatGPT has shown us, AI has evolved to mimic the capabilities of humans to a startling degree.
It is also helpful to recognize that the term AI encompasses various sub-fields, including machine learning, natural language processing, computer vision, etc. For the sake of simplicity, I will refer to all as “AI” but differentiate between the flavours of AI where appropriate to understand how it works and why it is the right tool for the job.
A Brief History
A very brief history of AI helpful to understand why it is so capable today and the current applications AI is best suited for vs. what areas AI still struggles in.
1950s: The start of the modern AI movement. Alan Turing publicly wonders if machines can think and creates his famous “Turing Test” to help determine if a computer has achieved a ‘human’ level of intelligence. The term’ artificial intelligence’ is coined, the first AI conference occurs, and the first “AI” software programs are developed.
1960s: The first development of ‘neural networks,’ an attempt to build software programs that mimic the architecture of our human brains and a fundamental design for future AI applications.
1980s: Advancements in computer processing technologies allow for advancements in AI. New technologies that enable neural networks to ‘learn’ through backpropagation are developed, further mimicking how the human brain learns.
1990s: AI development continues to be closely correlated with rapid increases in processing power available. IBM’s “Deep Blue” algorithm beats world chess champion Gary Kasparov.
2000 – 2010s: Explosion in development and interest in AI based on accessibility to large datasets and processing power, especially with the creation of commercially accessible ‘cloud’ storage and processing resources such as Amazon Web Services, Microsoft Azure, and Google Cloud, dramatically lowering the cost and cost of entry for training and deploying AI applications. Algorithms beat champions in Jeopardy and impressively in the complex game of Go. Image recognition technologies, such as convolutional neural networks, undergo rapid development allowing for the development of self-driving cars and other vision-focused applications.
2020s: Development of new techniques, including ‘transformers’, allow for the development of large-language-model (LLM) based applications such as Open Ais ChatGPT and Google Bard and an explosion in the use of ‘generative AI’. These applications not only effectively mimic human conversational styles, but they can also use their massive training databases to synthesize new content. Image-based generative AI applications also propagate rapidly, allowing new images to be synthesized via text prompts from an extensive database of previous imagery.
Click on the image below to enlarge:
There are a few key take aways from the history above.
1. Development of AI has been closely associated with advancements in computer processing, storage, and accessibility. AI algorithms require massive amounts of data and processing power to ‘learn’ and their development is closely related to the cost of both acquiring and analyzing the datasets required. Continued development of advanced storage and processing capabilities will unlock future AI advancements.
2. AI success to date has been a mix of attempting to mimic the known architecture of the human brain (e.g., foundation of neural networks with backpropagation for most AI applications), and by playing to the strengths of machines in providing structured and consistent data sets for ‘learning’.
3. In some specific instances / applications, AI algorithms now perform better than expert humans.
4. We are at an ‘inflection point’ where AI applications are moving from very specific (e.g., a program designed specifically to identify fraud in banking transactions) to more generalized (e.g. ChatGPT, a program that allows users to ask a wide range of queries from “build me an e-commerce site including required code” through to “create a short story about a puppy named Spot”).
AI is a toolset, not a specific application, and as such has potential in almost every business process. It has evolved from long standing mathematical methodologies such as statistics and probability theories, supercharged by access to today’s massive computing resources. However, understanding where AI has evolved from is important to understand where it has the most potential as a tool to unlock value for your business.
How is AI Transforming Business’ Today?
While AI applications exist for almost any business function imaginable, below is a small example of how AI is transforming business today.
Industrial Optimization
Using AI/Machine Learning, companies can analyze historical datasets to create models that optimize inputs across an impressive range of industrial processes. Especially when combined with modern Internet-of-things (IoT) sensors, AI can help optimize complex industrial processes like never before to unlock significant value for companies.
Preventative Maintenance
Like industrial optimization above, organizations can build probability models that predict the risk of maintenance failure, allowing for finely optimized maintenance programs and avoiding costly downtime.
Image Recognition
Using AI architectures such as Convolutional Neural Networks, applications can analyze images to learn and recognize objects and patterns. This has a wide range of applications, including using AI to scan medical images to identify patient issues, core logic for self-driving cars, recommending fertilizer and irrigation plans in agriculture, quality control in manufacturing, and more.
Fraud Prevention
AI applications can analyze massive and disparate datasets to identify subtle patterns and indicators of fraud. This supports not only the prevention of credit card or other financial fraud but also in identifying plagiarism in education and commercial applications, fake reviews, and other areas where fraud erodes trust and leads to financial and non-financial costs to companies and their clients.
Dynamic Pricing
AI’s ability to analyze massive and changing datasets allows it to generate effective pricing recommendations based on changing conditions. This can generate dynamic pricing for ride-share apps, parking, and fuel/energy stations, where changing conditions require a more responsive pricing strategy.
Chatbots
Using a combination of natural language algorithms, AI-driven chatbots have become ubiquitous, with the ability to answer many client and employee questions without requiring a human agent. With the development of large language models and generative AI applications such as ChatGPT, chatbots are becoming even more capable and difficult to differentiate between humans providing the same service.
Personal Assistants
Similar to the deployment of chatbots, Siri (Apple), Cortana (Microsoft), and Alexa (Google) are all examples of AI-driven applications that allow us to interact with technology using natural language prompts and conversations. It is the evolution towards a more ‘Star Trek’ future where we interact with our technology using verbal communication as much as physical inputs.
The applications above are only a tiny sample of how AI is leveraged in business today. It is a rapidly evolving field, and exciting new AI applications are being developed and released constantly – while this section may already need to be updated by the time you read it, some recent developments in the field include:
Generative AI
Generative AI (GenAI) leverages relatively new AI architectures to allow the algorithms to synthesize new content, analyzing massive amounts of training data. A key difference with GenAI applications is that while in the past, AI applications had to be trained, tuned, and focused on a relatively narrow application to be successful, the new GenAI applications are impressively flexible in their use.
ChatGPT and similar applications now generate computer code from a relatively small number of natural language prompts. This allows non-experts to write basic programs and for experts to supercharge their productivity, by optimizing code and focusing on challenging problems instead of spending their time on lower-value tasks. Dall-E, Stable Diffusion, and Midjourney allow users to generate a wide array of images from relatively simple text prompts – opening artistic generation and expression to those who were unable to create them using traditional or digital creative techniques before.
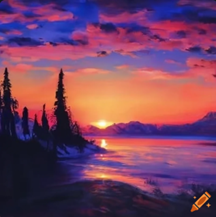
Figure 1: “Vivid impressionist painting of the pacific northwest at sunrise” generated via Craiyon.com.
GenAI is no substitute for human creativity and expert knowledge, but GenAI tools have allowed professionals in many fields to become significantly more efficient and productive. For example, it is likely that most of the corporate social media posts you see today leveraged GenAI tools in some capacity. It is also likely that many of your employees, contractors, and business partners are using GenAI in their services provided to you.
Business ‘Co-Pilots’
Based on GenAI technology, a range of business co-pilots are being released. For example, Microsoft Co-Pilot for Office is an emerging technology that could significantly increase the efficiency of many standard office processes.
From generating a spreadsheet from a basic text prompt to creating a PowerPoint ‘pitch deck’ based on previously transcribed chat messages and emails, we will see many time-consuming office administrative tasks where AI can generate an initial draft that can then be optimized and polished by a specialist focusing on higher value work.
Responsible AI
As the AI transformation impacts businesses, organizations, and institutions across the globe governments are under increasing pressure to develop effective regulations to ensure AI is a positive force and not a negative one. This is a massive challenge, considering the difference in velocity between innovation in AI and government processes. However, it is a critical one, and even while regulations are being developed an industry-driven responsible AI push is underway to provide guidelines and guardrails to AI development and deployment, minimizing the risk to your business when deploying AI applications. A key component of this course is to review the tenants of responsible AI and incorporate it into your AI Strategy.
Case Studies
One example of how AI adds value to organizations is the recent experience of AES.
AES is an international renewable energy company that is looking to drive cost savings and improve reliability by improving its ability to predict failures, optimize the energy output from its generation assets, and optimize load distribution.
A two-year AI transformation journey was launched by first focusing on developing and approving an AI strategy, including working with key partners to identify their highest-value use cases, which were;
1. Wind Turbine Predictive Maintenance
Wind turbines are complex machines operating in dynamic environments and require consistent maintenance to ensure optimal operation. This maintenance can require specialized and expensive equipment, and repairs can easily cost upwards of $100K per item. In their analysis, an ability to predict and plan essential repair work vs. being reactionary could save upwards of 60% on maintenance and AES now estimates it has already saved millions of dollars in maintenance costs using this AI-enabled process.
2. Energy Bidding Strategy for Hydro Plants
AES owns and operates numerous hydroelectric plants in deregulated power markets, requiring them to bid on a price for the power generated. Using AI algorithms, there is a significant opportunity to maximize the value of their generated power, and AES has deployed these algorithms for its hydro and natural gas generation facilities – however, due to commercial sensitivities, it has not disclosed how much extra revenue they expect to have made from the algorithms.
3. Smart Meters
The company leverages over one million digitally connected smart meters to monitor energy consumption. Using AI, they reduced the number of times a technician was sent unnecessarily to repair a meter, eliminating 3,000 non-essential “truck rolls” and generating an annual savings of ~$1M.
AES has now generated millions of dollars of value from its AI program and leverages more than 150 models across 85 use cases. More recent use cases within the company include wake steering for wind turbines and an enhanced vegetation management program that has reduced their Customer Average Interruption Duration Index (CAIDI) by 10% – a key operational performance metric for the industry.
In another similar example, Daimler Trucks Asia (DTA) partnered with Deloitte to identify and deploy an AI-based solution to “predict, detect, and remediate repairs”. After going through the critical processes required to identify and collect the necessary data, develop the AI application with support infrastructure, and deploy it, DTA reports that through this project, they expect to:
• Save $8 million in warranty costs during the first 24 months of the project and even more in recall costs.
• Predict and prioritize quality issues 13 months ahead of their previous process.
The examples above illustrate how it can add significant value even in the early stages of an AI transformation. Other companies, such as Netflix, rely on AI as a core strategic enabler – think of how its recommender engine is central to driving the user experience and maintaining and growing its subscriber base. AI is a massive value generator for companies that can leverage it strategically.
What is an AI Strategy?
An AI strategy provides the critical framework and ‘guardrails’ to guide an organization through the rapidly evolving AI transformation. It allows an organization to:
• Understand how AI, in general, is currently affecting your business and market sector.
• Identify the specific AI applications that your clients, partners, and employees are likely using today and how this impacts your business.
• Identify high-level business processes within your organization where AI can add significant value immediately.
• Generate a technology roadmap to estimate when AI development will add significant value to future business process areas.
• Ensure your organization has the internal structure to support the management and deployment of AI effectively.
• Develop the governance structure to deploy AI within a rapidly evolving regulatory environment effectively.
• Create critical targets and metrics to track the growing value of AI within your organization.
An AI strategy is a must for all businesses. This program will help guide you through creating this critical framework, allowing you to navigate the AI transformation confidently and allowing your business to proactively capture the immense value and opportunities AI unlocks instead of reacting ad hoc to the numerous changes AI is bringing to your industry.
Curriculum
AI Strategy – Part 1- Year 1
- Part 1 Month 1 Define Success
- Part 1 Month 2 AI Foundations
- Part 1 Month 3 Measured Value
- Part 1 Month 4 Strategic Structure
- Part 1 Month 5 AI as a Product
- Part 1 Month 6 Key Technologies
- Part 1 Month 7 Data Driven
- Part 1 Month 8 Generative AI
- Part 1 Month 9 Governance, Risks, and Responsible AI
- Part 1 Month 10 Organizational Change Management
- Part 1 Month 11 Sustainment and Scaling Up
- Part 1 Month 12 Action Plan
Program Objectives
The following list represents the Key Program Objectives (KPO) for the Appleton Greene AI Strategy corporate training program.
AI Strategy – Part 1- Year 1
- Part 1 Month 1 Define Success – Clearly define what success will look like at the end of the course and visualize the best possible outcome(s) for the participants and organization. This will occur by delving into why participants are taking the course in the first place and what challenges and perceived opportunities from AI have been explored in the organization to date.
- Part 1 Month 2 AI Foundations – Provide context and background surrounding AI/ML, with a focus on where it has been successfully deployed into organizations in the past. Understanding where AI/ML technologies have come from, as well as some of the recent key innovations in the space, will better enable participants to understand which opportunities and challenges this technology would be best suited for in their organization.
- Part 1 Month 3 Measured Value – Explore current methods to track and measure value, then review and identify an organizationally appropriate means of defining, measuring, and monitoring value generated from AI products within the organization.
- Part 1 Month 4 Strategic Structure – Organizational structures that increase the potential for successful AI deployment and integration are available. This workshop reviews some of the more common support structures used in organizations for AI Product development, deployment, and sustainment, including Centers of Excellence / Guilds and Partner-Driven models.
- Part 1 Month 5 AI as a Product – Pivot from a more conceptual conversation around AI into a specific overview of converting AI concepts into usable products for organizations. Review common structures used to successfully ‘productize’ AI in an organization.
- Part 1 Month 6 Key Technologies – Explore key technologies used in developing, delivering, and sustaining AI products. This section is dynamic, as AI-supporting tech is evolving so rapidly, but the focus is on larger, more enterprise-grade ecosystems such as Microsoft Azure, Google Cloud, and Amazon AWS to provide context on what key tools are likely already available for an organization to leverage for their AI practice.
- Part 1 Month 7 Data Driven – This workshop is dedicated to the most common challenge in successfully using and generating value from AI products…access to appropriate, timely, and trusted data. Reviewing common AI architectures, data issues, and potential solutions will help organizations get a head start on this critical and often (initially) overlooked vital enabler for their AI practice.
- Part 1 Month 8 Generative AI – Generative AI (GenAI), including well-known products such as Chat GPT, is such an impactful technology that an entire session will focus on understanding GenAI, typical applications in business today, and identifying where it has the highest transformative potential in your organization.
- Part 1 Month 9 Governance, Risks, and Responsible AI – Understanding the risks of using AI products in an organization is critical. Bias and other unintended outcomes can occur without proper design and governance of AI products, and organizations need to be aware of several national legislations in development and international standards.
- Part 1 Month 10 Organizational Change Management – Transformative efforts require careful planning and preparation to ensure acceptance into your organizational culture. An AI-focused organizational change management plan fosters clear communication, effective training, and embracing new technologies and processes, ensuring your AI transformation can achieve its vision.
- Part 1 Month 11 Sustainment and Scaling Up – Deployment of AI is only the start of the journey – a sustainment and scaling plan is required to ensure long-term value and adaptability as business needs evolve. It helps maintain AI system performance, enables continuous improvement, and prepares the organization to expand AI capabilities effectively and strategically.
- Part 1 Month 12 Action Plan – After completing the previous workshops, you have a clear vision of how to successfully deploy AI within your organization. It’s time to create a list of high-value AI use cases to focus on, develop an implementation/execution roadmap, and start your journey.
Methodology
AI Strategy
Throughout the program, we will be referencing several models for the successful implementation of AI programs, including the importance of alignment and communication using models such as Simon Sinek’s “Golden Circle” or using the “Plan-Do-Check-Act (PDCA)” Cycle during implementation. However, this program follows the following high-level methodology to set your organization up for success.
Phase 1: Build the Foundation
The first four sessions are about building a foundation of shared understanding and language for the rest of the journey. We dig into why you chose to take part in this course so that we can quantify what success looks like right at the start, which is critical to optimizing the positive impact AI can have on your organization.
A look at the history and evolution of AI will ensure we understand how the historical development of AI products has led to the capabilities, benefits, and risks of AI applications today. Reviewing common means of measuring value through metrics will help us build the guardrails necessary to ensure AI deployments stay focus on value, and not just technology for technologies sake.
Finally, we will explore different strategic structures available for organizational AI teams to determine which is fits best with your organizational culture and goals, including internal, centre-of-excellence, and partner-focused structures.
Phase 2: Sharpen the Tools
The following six sessions focus on empowering your team with the tools and knowledge required to deploy and integrate AI into your business effectively. Discussing “AI as a Product” ensures that we look at AI through the lens of a powerful tool for your business instead of as a hypothetical or academic concept discussed in the abstract. We will review successful people, process, and technology AI structures, identify the most appropriate for your organization, and dig into cutting-edge tools such as generative AI (GenAI), which are transforming entire market sectors today.
This phase includes reviewing specific roles that enable successful AI integration, strategic organizational support structures including Centers of Excellence opportunities, key technologies including cloud services to support AI deployments, “Machine Learning Operations (MLOps)” and other industry standard operational models, a strong focus on the critical importance of trusted data, and a review of governance and risk mitigation strategies for successful AI deployment including Responsible AI principles and practices.
Phase 3: Ready for Launch
Now that we know the essential tools and structures required to deploy a successful AI practice and clearly understand what success looks like for your specific organization, we are ready to build a supercharged AI strategic plan appropriate and tailored to your business.
The final four sessions focus on developing your AI strategic plan, including a high-level implementation roadmap and a retrospective on how far you have come throughout the program. You will have an AI action plan ready to execute at the end of the final session.
Industries
This service is primarily available to the following industry sectors:
Energy (Oil & Gas / Utilities)
The energy sector is a fundamental pillar of modern society, encompassing the production, distribution, and consumption of various forms of energy that power our daily lives. It is pivotal in driving economic growth, enabling technological advancements, and supporting our basic needs. It relies on a diverse array of energy sources, broadly categorized as follows:
Fossil Fuels – These include coal, oil, and natural gas. They have dominated the energy sector in the recent past and continue to be significant contributors to global energy production.
Renewable Energy – This category comprises sources like solar, wind, hydroelectric, geothermal, and biomass energy. The adoption of renewable energy has been steadily growing due to environmental concerns and technological advancements.
Nuclear Energy – Nuclear power plants generate electricity through controlled nuclear reactions. Although controversial due to safety concerns, nuclear energy remains a significant part of the energy mix in some countries. As well, investment in prototype fusion technology is increasing, raising the possibility of a new nuclear based energy source in the future.
This broad diversity of energy sources is one of the challenges when looking at the ‘energy sector’ – it is a massive sector from an economic and industrial perspective. However, it can be helpful to look at it from the standpoint of production, distribution, and consumption:
Energy Production – Involves energy extraction, conversion, and generation from various sources. This can range from drilling for oil and natural gas to harnessing wind energy with turbines. Each energy source requires distinct infrastructure and technologies for extraction and conversion.
Energy Distribution – Energy must be efficiently transported from production sites to end-users. This is achieved through an intricate network of pipelines, transmission lines, and distribution grids. The development of smart grids and digital technologies has improved the reliability and flexibility of energy distribution.
Energy Consumption – Energy consumption occurs in various sectors, including residential, commercial, industrial, and transportation. These sectors have different energy needs and efficiencies. Energy-efficient technologies and practices are crucial for reducing consumption and minimizing environmental impacts.
Within these broad categories, we can dig deeper into specifics. For example, in the oil and gas industry, it is helpful to compartmentalize the entire process flow into sub-sectors such as “upstream” (the exploration, drilling, and extraction of the resource), “midstream” (infrastructure designed to transport and store oil and gas before refinement into processed fuels), and “downstream” (refining crude oil and natural gas into gasoline, diesel, propane, and other finished products to the point of sale to customers). These sectors have unique opportunities and challenges throughout the current energy transformation.
AI and the Energy Sector
The energy sector is at the forefront of global innovation, undergoing significant transformation driven by technological advancements. Recent years have witnessed a surge in the adoption of Artificial Intelligence (AI) and other cutting-edge technologies that are revolutionizing the industry. Some of the specific applications of AI in the energy industry include:
Enhanced Efficiency and Resource Optimization – Artificial Intelligence has enabled the energy sector to optimize its operations and resources. Through predictive analytics and machine learning algorithms, AI systems can accurately forecast energy demand patterns, allowing utilities to allocate resources more efficiently, reducing waste and minimizing costs. AI-powered predictive maintenance has also become a critical tool, helping companies identify and address equipment issues before they lead to costly breakdowns.
Smart Grids and Energy Management – Integrating AI has supported the development of smart grids. These grids use AI algorithms to manage real-time electricity distribution, making the energy supply more reliable and resilient. Smart grids also enable demand-side management, allowing consumers to control their energy consumption intelligently. This, in turn, reduces energy waste and promotes sustainability.
Renewable Energy Integration – The growth of renewable energy sources, such as solar and wind, has been accelerated by AI-driven innovations. AI helps overcome the intermittent nature of renewables by forecasting energy generation and optimizing energy storage solutions. For instance, AI can predict weather conditions and adjust energy production and storage accordingly.
Energy Trading and Market Optimization – AI has great potential for energy trading and market operations. AI algorithms analyze vast amounts of data from various sources, including weather forecasts, historical energy prices, and demand patterns, to make real-time trading recommendations. Automated trading systems can also enhance risk management, ensuring energy companies can make more informed decisions in a dynamic marketplace.
The energy sector is undergoing a profound transformation, with technological innovations, particularly Artificial Intelligence, playing a pivotal role. These innovations have enhanced the efficiency and reliability of energy production and distribution and contributed significantly to the global push for sustainability. As AI advances, it will unlock even more potential in the energy sector, making it more resilient, profitable, and adaptable to future challenges.
Architecture, Engineering, and Construction (AEC)
The Architecture, Engineering, and Construction (AEC) sector is a multifaceted industry that plays a pivotal role in shaping the built environment we live in today. The three foundational disciplines work together to imagine, design, and then build the physical world we enjoy.
Architects are responsible for conceptualizing and designing buildings and structures that are functional and aesthetically pleasing. They consider factors like sustainability, safety, and user experience while incorporating innovative designs and materials to meet the evolving needs of society.
Engineering involves various disciplines, including civil, structural, mechanical, and electrical engineering. Engineers provide the technical expertise required to transform architectural plans into reality. They ensure that buildings and infrastructure are safe efficient and comply with relevant codes and regulations.
Construction is the physical realization of architectural and engineering plans. Construction companies and workers are responsible for bringing designs to life, managing resources, labor, and schedules, and ensuring that projects are completed within budget and to specifications. This aspect of the AEC sector includes residential, commercial, industrial, and infrastructure projects.
Some key trends and challenges in the AEC sector include:
Sustainability and Green Building – There is a growing emphasis on sustainability and green building practices. For example, the Leadership in Energy and Environmental Design (LEED) standards provide an internationally recognized certification structure for sustainability in the sector.
Building Information Modeling (BIM) – BIM technology has revolutionized the AEC sector by enabling multidisciplinary teams to collaborate effectively. BIM allows stakeholders to create digital representations of buildings and infrastructure, improving project coordination, reducing errors, and enhancing decision-making throughout the project lifecycle.
Digitalization and Automation – The AEC sector is transforming digitally by adopting drones, robotics, and AI technologies. These innovations enhance efficiency, accuracy, and safety in construction and project management. Digital tools also facilitate remote collaboration, a necessity during the COVID-19 pandemic.
Regulatory Complexity – Navigating the complex web of building codes, permits, and regulations can be challenging for AEC professionals. Staying up-to-date with evolving standards is crucial to avoid delays and legal complications.
Skilled Labor Shortages – The AEC sector, including architects, engineers, and construction workers, faces skilled labor shortages. This scarcity can lead to project delays and increased labor costs.
Cost, Schedule and Quality Pressures – Projects in the AEC sector can face cost, schedule, and quality issues due to unforeseen challenges, weather conditions, or supply chain disruptions. Effective project management is essential to mitigate these issues.
AI in AEC
AI is a transformative force in the AEC sector, offering numerous advantages across various phases of projects. AI technologies have the potential to streamline processes, enhance decision-making, and improve the efficiency and sustainability of construction projects. Some examples of how AI can benefit the AEC sector include:
Design Optimization – AI-driven algorithms can analyze vast amounts of data, including historical project data and environmental factors, to generate optimized design solutions – leading to more sustainable and cost-effective architectural designs.
Building Information Modeling (BIM) Enhancements – BIM tools enhanced with AI can facilitate better collaboration among multidisciplinary teams by automatically detecting clashes and inconsistencies in project plans. Additionally, AI-driven BIM can simulate and predict the performance of buildings, helping architects and engineers refine designs for efficiency and safety.
Predictive Maintenance – AI-enabled sensors and data analytics can monitor the health and performance of infrastructure and buildings in real time. AI can predict maintenance needs by analyzing sensor data, reducing downtime, and preventing costly repairs.
Project Management – AI-powered project management tools can optimize schedules and resource allocation, predict potential delays, and offer solutions to mitigate risks. These tools can also improve communication and coordination among project stakeholders.
Safety and Risk Mitigation – AI can enhance safety on construction sites by monitoring compliance issues and identifying potential hazards. It can also analyze data to assess project risks and suggest proactive risk mitigation strategies.
Supply Chain Optimization – AI algorithms can help optimize supply chain management by predicting material requirements, tracking inventory levels, and optimizing procurement processes – reducing waste, lowering costs, and ensuring timely material deliveries.
Drone and Robotics Integration – Drones equipped with AI can provide aerial surveys, monitor progress, and generate detailed site maps. AI-powered robotics can be used for repetitive and labor-intensive tasks, reducing labour costs and improving safety.
Energy Efficiency – AI-driven building management systems can continuously monitor and adjust heating, cooling, lighting, and other systems to optimize energy consumption, resulting in significant cost savings and reduced environmental impact.
Natural Language Processing (NLP) for Documentation – NLP technology can streamline document management and communication by automatically extracting and organizing information from various documents, emails, and reports, reducing administrative burdens.
AI offers significant benefits to the Architecture, Engineering, and Construction sector. It can revolutionize how projects are planned, designed, executed, and maintained, ultimately leading to more efficient, sustainable, and cost-effective construction processes.
Telecommunications
The telecommunications sector is a critical component of the global economy, connecting people, businesses, and governments worldwide. It encompasses a wide range of technologies, services, and infrastructure that enable the transmission of voice, data, and video over long distances.
It has a rich history that dates back to the invention of the telegraph in the 19th century. Over the years, it has witnessed significant technological advancements that have reshaped how people communicate. Notable milestones include the invention of the telephone by Alexander Graham Bell in 1876, the development of the transistor in the 1940s, and the advent of the internet in the late 20th century. These innovations have paved the way for the modern telecommunications landscape, characterized by a convergence of voice, data, and multimedia services. It can be broadly grouped into the following categories:
Infrastructure – The telecommunications sector relies heavily on a vast network infrastructure, including fiber optic cables, satellite systems, cellular towers, and data centers. These physical assets form the backbone of global communication networks.
Service Providers – Telecom service providers, both wired and wireless, offer a wide range of services to consumers and businesses. These include voice calls, internet access, television broadcasting, and cloud-based services.
Equipment Manufacturers – Companies like Cisco, Huawei, and Nokia produce the hardware and equipment needed to construct and maintain telecommunications networks.
This key sector is experiencing rapid transformation from a technological, regulatory, and consumer perspective due to the following factors:
Technological Advancements – Emerging technologies like 5G, the Internet of Things (IoT), and edge computing are transforming the sector, offering faster speeds, lower latency, and new opportunities for service providers.
Security – Cybersecurity threats pose a significant risk to network operators and users. Protecting data and infrastructure from cyberattacks is an ongoing concern.
Emergence of 5G and Spectrum Allocation – As the demand for wireless services grows, the spectrum allocation for 4G and 5G networks has become a vital issue for governments and regulatory bodies to manage. The deployment of 5G networks will bring faster speeds and low latency, enabling new applications such as augmented reality, autonomous vehicles, and smart cities. However, the cost of deploying next-generation 5G networks is causing M&A activity in the sector and pressure on balance sheets.
Consumer Expectations – With the proliferation of smartphones and high-speed internet, consumers now expect seamless connectivity, high-quality streaming, and personalized services. Meeting these demands is a constant challenge.
IoT Growth – The Internet of Things is experiencing exponential growth, connecting billions of devices and enabling innovations in healthcare, agriculture, and manufacturing. However, this growth is pressuring telecommunications networks and helping to drive the deployment of expensive 5G infrastructure.
Edge Computing – Edge computing will become more prevalent, reducing latency and enabling real-time data processing, particularly for applications like autonomous vehicles and industrial automation.
Satellite Internet – Satellite-based internet providers like SpaceX’s Starlink are expanding their reach, bringing high-speed internet to remote and underserved areas and challenging traditional wireline and wireless providers.
AI in Telecommunications
AI supports telecommunications by offering solutions that enhance network efficiency, customer experience, and operational capabilities. Some examples of these solutions include:
Network Optimization – AI algorithms can analyze vast amounts of network data in real time to identify and rectify network congestion, reduce downtime, and improve overall network performance. This proactive approach ensures a smoother user experience and helps telecom providers meet the increasing demands of data-hungry applications.
Predictive Maintenance – By analyzing data from sensors and equipment, AI algorithms can forecast equipment failures before they occur – minimizing downtime, reducing maintenance costs, and enhancing the reliability of telecom networks.
Customer Service and Support – Chatbots and virtual assistants can respond immediately to customer inquiries, troubleshoot common issues, and even assist with billing and account management. This improves customer satisfaction and reduces the workload on human customer support agents.
Fraud Detection and Security – AI safeguards telecom networks against fraud and security threats. Machine learning models can analyze patterns of fraudulent activities, such as SIM card cloning or call detail record (CDR) anomalies, and trigger alerts or preventative measures. AI-driven security solutions help protect customer data and maintain the integrity of telecom networks.
Personalized Services – Telecom companies can use AI applications to offer personalized services and recommendations to customers. By analyzing user data, AI algorithms can tailor service plans and content suggestions, increasing customer engagement and loyalty.
Network Security – Cybersecurity solutions leveraging AI provide next-generation abilities to proactively identify and respond to threats, such as DDoS attacks and malware infiltration, protecting the network infrastructure and user data.
AI is a transformative force in the telecommunications sector, offering solutions that enhance network performance, customer satisfaction, and operational efficiency. As the demand for high-speed connectivity and digital services continues to grow, telecom companies that embrace AI technologies are better positioned to thrive in an increasingly competitive and dynamic industry. AI-driven insights and automation will be integral in delivering superior services and maintaining the reliability and security of telecommunications networks.
Technology
The technology sector encompasses many companies and sub-industries that develop, produce, and distribute technological products and services. It is an incredibly dynamic sector, with the rate of innovation continuing to accelerate. While it is challenging to paint the technology sector in broad strokes, some high-level components include:
Semiconductors – Semiconductor manufacturers produce the chips and microprocessors that power electronic devices. The dominant players are Intel, AMD, and Taiwan Semiconductor Manufacturing Company (TSMC).
Hardware – This includes the manufacturing and distribution of electronic devices such as smartphones, computers, servers, and networking equipment. Cisco, Intel, HP, Apple, Samsung, and Huawei are examples of companies in this space.
Software – Software companies design, develop, and distribute applications, operating systems, and software solutions. Notable consumer examples include Microsoft, Electronic Arts, and Adobe.
Internet and E-commerce – Companies in this category focus on online services, e-commerce platforms, and Internet-related technologies. Amazon, Google, and Alibaba are leaders in this sector.
Cloud Computing – Cloud providers like Amazon Web Services (AWS), Microsoft Azure, and Google Cloud offer infrastructure, platforms, and software as services, enabling businesses to scale and innovate more efficiently.
Artificial Intelligence and Machine Learning – AI and ML companies develop algorithms and technologies that enable machines to learn and make intelligent decisions. OpenAI, NVIDIA, Google, and IBM are influential in this domain, however it is rapidly developing with new startups impacting the space and established technology players investing significantly.
Some high-level trends currently impacting the sector include:
AI and Automation – AI and robotics are transforming the industry. From coding ‘co-pilots’ to AI-powered manufacturing, AI is rapidly reducing the cost from idea to profitable company and accelerating mature technology companies.
Remote Work and Collaboration Tools – The COVID-19 pandemic accelerated the adoption of remote work and collaboration tools, leading to increased demand for video conferencing (Zoom, Microsoft Teams), project management (Trello, Asana), and communication (Slack, Discord) platforms. While many organizations have moved away from an entirely remote workforce, the hybrid work model remains popular, requiring the continued support and development of successful remote work and collaboration infrastructure.
5G Technology – The rollout of 5G networks promises faster and more reliable wireless connectivity, enabling advancements in autonomous vehicles, IoT, augmented reality, and virtual reality. This telecommunications backbone will enable many of the promised AI-driven future innovations.
Cybersecurity – With the rise in cyber threats, companies invest heavily in cybersecurity solutions to protect their data and systems. Leading cybersecurity firms include Palo Alto Networks, CrowdStrike, and Fortinet.
Space Technology – The space tech sector is proliferating, with companies like SpaceX, Blue Origin, and satellite internet providers seeking to expand access to space and satellite-based services challenging traditional telecommunications models.
The sector is also navigating some high-level challenges, including:
Regulation and Privacy – As technology advances, concerns about data privacy, cybersecurity, and antitrust issues have increased regulatory scrutiny and legal challenges for tech companies.
Supply Chain Disruptions – The global supply chain disruptions caused by events like the COVID-19 pandemic and geopolitical tensions have impacted the availability of semiconductor chips and other critical components. However, these disruptions do appear to be subsiding.
Talent Shortage – The technology sector needs more skilled professionals, particularly in AI, data science, and cybersecurity.
Ethical Concerns – The ethical use of technology, especially AI, has become a significant concern, with discussions about bias, fairness, and responsible AI gaining prominence.
AI in Technology
AI is a foundational innovation for the technology sector. It will impact every technology business area, and analyzing AI’s impact is best suited at a company-by-company level. However, at a macro-level, AI is transforming technology by:
Product Development and Innovation – AI accelerates the development of cutting-edge technology products and solutions. It aids in automating design processes, predicting market trends, and improving research and development efforts, ultimately leading to faster innovation cycles.
Automation and Efficiency – AI-powered automation streamlines various processes within tech companies, from data entry and customer support to software testing and cybersecurity. This results in cost savings and improved operational efficiency.
Data Analysis and Insights – AI excels at processing and analyzing vast datasets, enabling technology companies to derive valuable insights for informed decision-making. Whether understanding user behavior or optimizing supply chains, AI enhances data-driven strategies.
Enhanced User Experiences – Personalization and recommendation engines powered by AI create tailored experiences for tech product users, leading to higher customer satisfaction and increased user engagement.
Cybersecurity – AI is pivotal in detecting and mitigating cyber threats in real time. It can analyze network traffic patterns to identify anomalies and protect sensitive data from evolving security threats.
Natural Language Processing (NLP) – AI-driven NLP technology enhances communication and interaction with technology products. Voice assistants, chatbots, and language translation services are prime examples of NLP applications.
Supply Chain Optimization – AI optimizes the supply chain by predicting demand fluctuations, managing inventory levels, reducing delivery times, ensuring a steady flow of essential components, and reducing operational costs.
Market Analysis and Competitive Intelligence – AI can gather and analyze market data, competitor strategies, and consumer sentiment, aiding tech companies in making strategic decisions.
Generative AI (GenAI) – While GenAI tools will be integrated into all categories above, it deserves a special mention. An ecosystem of GenAI tools is being developed and released to provide a new experience from a support perspective, allowing access to new capabilities previously unavailable from traditional software products.
AI is a transformative force in the technology sector, permeating every aspect of operations from product development to customer service. As AI advances, technology companies that harness its capabilities will stay at the forefront of innovation, ensuring their continued relevance and competitiveness in a rapidly evolving industry.
Agriculture
Agriculture is a cornerstone of human civilization, providing food, raw materials, and livelihoods for billions of people worldwide. It encompasses various activities, from crop cultivation and livestock farming to forestry and fisheries. Agriculture is fundamental to global food security, producing the world’s food supply, including staples like rice, wheat, maize, and numerous fruits and vegetables.
Agriculture also contributes significantly to the economies of many countries. It provides employment opportunities for a substantial portion of the global workforce, particularly in developing nations. Furthermore, it serves as the backbone of most rural economies. Beyond food, agriculture produces raw materials for various industries, such as cotton for textiles, timber for construction, and oilseeds for biofuels and industrial processes. From a sustainability perspective, the sector has a substantial environmental footprint, influencing land use, water resources, and biodiversity. Sustainable farming practices are rapidly being identified and developed to ensure food security in a dynamic world.
It is also important to recognize how innovative and technologically advanced agriculture has become, especially in developed nations. Some key recent innovations include:
Precision Agriculture – Precision agriculture utilizes a suite of tools such as GPS, on-machine sensors, and drones to optimize resource use, reduce waste, and increase productivity.
Biotechnology – Genetic engineering and biotechnology have led to the development of genetically modified (GM) crops customized for yield, pest, and climate-resistant traits.
Sustainable Practices – Organic farming, agroforestry, and permaculture are gaining popularity for farmers who prefer to avoid GM or chemical-intensive practices. They prioritize soil health, biodiversity, and reduced environmental impact.
Vertical Farming – Urban agriculture, including vertical farming and hydroponics, allows for year-round, resource-efficient cultivation of crops in controlled environments, reducing the need for extensive land areas. It is highly technology-dependent and has recently seen a surge of interest as the supporting technology develops.
Blockchain and Traceability – Blockchain technology enables transparent supply chains and product traceability, addressing food safety and authenticity concerns.
Current trends facing the sector include:
Digital Agriculture – Integrating digital technologies like artificial intelligence, big data, and the Internet of Things (IoT) will continue revolutionizing agriculture, enabling data-driven decision-making and precision farming.
Agri-Tech Startups – The agriculture sector is witnessing a surge in agri-tech startups developing innovative solutions to address various challenges, from crop monitoring to market access for smallholder farmers.
Sustainability – Finding the balance between the need for increased production and environmental stewardship is a crucial challenge. Regenerative agriculture, carbon sequestration, and improved resource management will play central roles.
International Cooperation – Global challenges like climate change and food security require international collaboration. Agreements and initiatives such as the Paris Agreement and the Sustainable Development Goals (SDGs) highlight the importance of coordinated efforts.
AI in Agriculture
AI is revolutionizing the management of farming and food production. Key innovations include:
Precision Agriculture – AI enables Precision Agriculture, which uses data and technology to optimize resource allocation and farming practices. Farmers can collect data on soil quality, weather conditions, and crop health, allowing for precise planting, irrigation, and fertilization. This not only increases yields but also reduces resource wastage and environmental impact.
Crop Monitoring and Disease Detection – AI and machine learning algorithms can analyze satellite imagery and drone data to monitor crop health and detect diseases and pests early. This proactive approach enables farmers to take timely action, minimize crop losses, and reduce the need for pesticides.
Yield Prediction and Crop Management – AI models can predict crop yields based on historical data, weather forecasts, and real-time field data. This information helps farmers optimize harvest timing and plan for storage and distribution, improving overall supply chain efficiency.
Autonomous Farming – AI-powered autonomous vehicles and robots are becoming integral to modern agriculture. These machines can perform tasks such as planting, harvesting, and weeding with high precision, reducing labor costs and the need for manual intervention.
Livestock Monitoring – AI-based systems can monitor the health and behavior of livestock using sensors and cameras, helping farmers identify issues early, improve animal welfare, and optimize breeding and feeding practices.
Climate Resilience – AI models can provide insights into climate change impacts on agriculture, helping farmers adapt to shifting weather patterns and make informed decisions about crop choices and water management.
Market Forecasting – AI-driven market analysis can provide farmers valuable insights into market trends, pricing, and consumer preferences. This information enables better decision-making regarding crop selection and marketing strategies.
Supply Chain Optimization -AI can optimize the agricultural supply chain by predicting demand, managing inventory, optimizing logistics, reducing food waste, ensuring timely deliveries, and improving the efficiency of food distribution systems.
Artificial Intelligence has the potential to transform the agriculture sector into a more efficient, sustainable, and resilient industry. By harnessing the power of AI, farmers and stakeholders can optimize resource usage, improve crop and livestock management, and respond effectively to the challenges of a changing climate and growing global food demand. As AI technologies advance, their role in agriculture will only become more significant, contributing to the sector’s growth and sustainability.
Locations
This service is primarily available within the following locations:
Calgary, AB
Calgary, nestled in the foothills of the beautiful Canadian Rockies, is a significant economic hub in Canada, primarily driven by its strong presence in the energy sector – particularly oil and natural gas. In 2019, Calgary’s Gross Domestic Product (GDP) was approximately $111 billion (CAD), contributing substantially to the Canadian economy.
Some major corporations that have a significant presence in the city include Suncor Energy, Canadian Natural Resources Limited (CNRL), Husky Energy (now part of Cenovus Energy), TransCanada Corporation (now known as TC Energy), and Enbridge Inc. These companies play pivotal roles in Canada’s energy industry, and their performance directly impacts Calgary’s economic health. However, Calgary does host a number of non-energy related companies such as WestJet, Canadian Pacific Kansas City Railway, and Shaw Communications (now part of Rogers) – and has the second-highest number of head offices in Canada. Calgary has also been growing its technology and financial services sector, contributing to its economic resilience, and includes recent tech ‘unicorns’ including Benevity and Neo Financial.
It has been successful in its goal of attracting businesses of all sectors with its business-friendly climate, entrepreneurial spirit, and consistently high international ‘livability’ scores – recently scoring third in the Economist’s annual ranking of global cities to live in. Fueled by its strong foundation in fossil fuel and renewable-based energy, proximity to Banff and the world-famous Canadian Rockies, and friendly, entrepreneurial spirit, Calgary is a city constantly re-imagining itself for the future.
AI in Calgary
Calgary is developing an AI ecosystem that includes several key companies and organizations driving innovation in artificial intelligence. The city’s AI sector benefits from the presence of top-tier educational institutions and a supportive tech community. Notable organizations include AltaML, a fast-growing machine learning and AI company that collaborates with businesses across various industries to develop AI solutions.
Calgary also hosts Amii (Alberta Machine Intelligence Institute), dedicated to advancing AI research and application, fostering collaborations, and providing resources for AI-driven projects. Another prominent player is Benevity, which employs AI to enhance workplace giving and corporate social responsibility programs. Additionally, the University of Calgary’s AI research and innovation initiatives contribute significantly to the city’s AI landscape, making Calgary a promising hub for AI-driven advancements in various fields.
Vancouver, BC
Located on the Pacific coast, Vancouver is the largest city in Western Canada and the third largest in the country. It has flourished as the key Pacific trading hub and has grown into Canada’s largest port, bringing in over $172 billion worth of trade with over 160 countries annually, and has hosted several international events – including the 1986 Expo and 2010 Winter Olympic Games.
Its strategic location and stunning natural setting have attracted companies from various industries. It is home to many of Canada’s forestry and mining companies and global’ lifestyle brands,’ including Lululemon, Arc’teryx, and Nature’s Path Foods. TELUS, one of Canada’s largest integrated telecommunications firms, is headquartered in Vancouver. Vancouver has also grown and attracted diverse technology companies, including Electronic Arts and General Fusion – acting as Canada’s proxy for San Fransico and Seattle.
Vancouver also has a vibrant film industry, being North America’s 3rd most prominent film and TV production center, supporting approximately 20,000 jobs in the space and supports a booming tourism industry, with over 10.3 million people visiting Vancouver in 2017. With a 2017 GDP of ~$137 billion and a growth rate of 4.5%, it continues to be a major economic center for Canada.
Vancouver is poised to leverage its diverse economy to drive innovation across various sectors. Successful technology start-ups continue to grow along with a foundation of traditional resource-based companies and a thriving real estate sector. The city is focusing on developing more housing to help new workers move to Vancouver, address cost-of-living concerns, and further develop the metro transit network. It continues evolving as a global city and an attractive place to live and work – ranking 5th on the Economist’s 2023 global livability rankings.
AI in Vancouver
Vancouver has swiftly emerged as a burgeoning AI hub on Canada’s west coast, driven by a combination of world-class research institutions and innovative tech companies. The University of British Columbia (UBC) and Simon Fraser University are at the forefront of AI research, particularly in machine learning and computer vision as is British Columbia Institute of Technology’s (BCIT) Natural Language Processing (NLP) Group.
Key AI companies, including D-Wave Systems, have made Vancouver their headquarters, focusing on quantum computing and machine learning. As the AI sector in Vancouver continues to expand, the city is attracting top talent and fostering an environment ripe for AI innovation and collaboration.
Toronto, ON
Toronto is the capital city of the province of Ontario and Canada’s largest metropolis, with a population of more than $6M. Canada’s financial capital contributes 20% of the national GDP and is a major North American and global financial and business centre. Its strategic location allows for quick access to major eastern North American centers and has served as the business center of Canada since the country’s founding.
Toronto supports various industries, including finance, technology, healthcare, education, and manufacturing. The city’s economic strength is characterized by its stability, diverse talent pool, and vibrant business ecosystem. At the core of its economic strength lies its gravity in the finance and banking sector as Canada’s financial capital, with major institutions like the Royal Bank of Canada (RBC), Toronto-Dominion Bank (TD), and Scotiabank headquartered in the city. The Toronto Stock Exchange (TSX) is one of the world’s largest stock exchanges.
The city has emerged as a global tech hub, driven by a burgeoning startup ecosystem, world-class research institutions like the University of Toronto, and the presence of tech giants like Shopify and Amazon Web Services. Artificial intelligence, fintech, and health tech are thriving sectors. Toronto is a leader in healthcare and biotechnology, with globally recognized institutions such as the University Health Network (UHN) and Sunnybrook Health Sciences Centre. The city hosts numerous pharmaceutical and biotech companies, contributing to medical research and innovation advances.
Toronto is also proud of its multiculturalism and strong social foundation – hosting a multitude of cultural events throughout the year and for pride in its sports teams, including the Toronto Maple Leafs (hockey), Blue Jays (Baseball), and Raptors (basketball).
AI and Toronto
Toronto has rapidly emerged as a prominent global AI player, attracting attention for its cutting-edge research and innovative AI companies. Anchored by the University of Toronto, the city’s thriving AI ecosystem is renowned for its contributions to deep learning and neural networks. One of the world’s most influential AI research labs, the Vector Institute for Artificial Intelligence, is also based in Toronto.
The city’s key AI companies and organizations include industry leaders like Element AI (now part of ServiceNow), an AI solutions provider, and Borealis AI, RBC’s research institute focused on AI innovation. Toronto’s AI sector continues to grow, fostering collaboration between academia and industry and making it a top destination for AI talent and innovation.
Houston, TX
With over $7 million people living in the greater Houston area, a 2023 GDP of over half a trillion dollars, and the second largest number of Fortune 500 companies (behind only New York), Houston, TX, is an economic powerhouse and is often referred to as the “Space City” and the “Energy Capital of the World.”
While historically rooted in the energy sector, particularly oil and gas, the city has evolved into a multifaceted economic powerhouse. Today, Houston’s economic mix encompasses several core sectors, such as energy – home to industry giants like ExxonMobil, Chevron, and ConocoPhillips – the city plays a pivotal role in global energy production and distribution.
Furthermore, Houston boasts the world’s largest medical complex, the Texas Medical Center, housing renowned institutions like the MD Anderson Cancer Center and Baylor College of Medicine. This healthcare and biotechnology hub drives medical innovation and contributes significantly to the local economy.
The city is also an essential space and aerospace hub. NASA’s Johnson Space Center positions Houston as a space exploration and research center. The city’s aerospace industry has led to a burgeoning aerospace manufacturing sector, with companies such as Boeing and Lockheed Martin making substantial economic contributions.
Houston’s technology sector is also rising, attracting startups and tech companies. Initiatives like the Houston Technology Center and the Houston Exponential incubator foster entrepreneurship and innovation, ensuring a healthy start-up ecosystem.
AI in Houston
Notable companies and organizations from an AI perspective is the University of Houston’s Artificial Intelligence Research Institute, a leading research center dedicated to AI innovations and applications in various fields.
The Houston AI and Machine Learning Meetup Group is a vibrant community for professionals and enthusiasts to collaborate and share knowledge in AI and machine learning. Houston also hosts AI-focused startups like Decisio Health, which specializes in clinical decision support systems, and Axiom Space, which uses AI to enhance space exploration capabilities. These organizations and companies exemplify Houston’s commitment to harnessing the power of AI for innovation and growth in various sectors, from healthcare to aerospace and beyond.
Seattle, WA
Located in the picturesque American Pacific Northwest, this city of ~4 million has a rich economic and cultural history. The technology sector is at the forefront of its diverse local economy, anchored by giants like Amazon and Microsoft. Amazon, one of the world’s largest e-commerce and cloud computing companies, was born in a Bellevue garage in 1994 and has since become an integral part of Seattle’s identity. Microsoft, founded by Bill Gates and Paul Allen, played a pivotal role in shaping the city’s tech prowess. These tech giants have attracted a wealth of talent and investment, fostering a thriving startup ecosystem that continues to push the boundaries of innovation.
Beyond technology, Seattle boasts a robust maritime industry, with the Port of Seattle serving as a vital gateway for international trade and commerce. The city’s aerospace sector, led by Boeing, has a storied history and remains a significant player in the global aviation industry. Seattle’s healthcare and life sciences sector, with institutions like the Fred Hutchinson Cancer Research Center and the University of Washington’s medical research facilities, contributes significantly to medical advancements and innovation.
The city is also known for its retail sector, largely thanks to retail giants like Starbucks and Nordstrom, which have roots in Seattle and have achieved global recognition. Furthermore, Seattle’s thriving arts and culture scene, including music, film, and gaming, is integral to the city’s economy, attracting creative talent and tourism dollars.
These corporate powerhouses and numerous startups and research institutions continue to attract top talent, foster entrepreneurship, and drive further economic growth. Notable startups include Zillow (real estate), Rover (pets), Convoy (logistics), and Auth0 (ID and Access Management). Additionally, Seattle’s thriving maritime industry, led by the Port of Seattle, plays a crucial role in international trade and commerce. The city’s commitment to sustainability and green initiatives further positions it as a leader in clean technology and environmental innovation.
AI in Seattle
With tech giants including Microsoft and Amazon based in the greater Seattle area, it is a crucial epicenter for AI, and primarily commercial AI, development. Many of the core AI-based innovations being used in companies today are through the cloud services of either Microsoft or Amazon with theoretical concepts converted into a commercial reality within this region.
The Allen Institute for AI (AI2), founded by Microsoft co-founder Paul Allen, is at the forefront of AI research, focusing on natural language understanding and machine learning advancements. Furthermore, Xnor.ai, a Seattle-based startup, gained recognition for its innovations in edge AI, particularly in computer vision. Seattle’s thriving tech ecosystem provides a fertile ground for AI research and innovation, making it a prime location for organizations and startups at the cutting edge of artificial intelligence.
Program Benefits
Operations
- Task Automation
- Predictive Maintenance
- Streamlined Processes
- Improved Accuracy
- Process Efficiency
- Risk Management
- Enhanced Reporting
- Increased Capacity
- Reduced Outages
- Improved Awareness
Marketing
- Customer Experience
- Partner Experience
- Opportunity Discovery
- Omnichannel Strategy
- Bespoke Campaigns
- Rapid Insights
- Funding Focus
- New Opportunities
- Success Tracking
- Brand Awareness
Finance
- Improved Reporting
- Risk Management
- Benefits Realization
- Anomaly Identification
- Expense Monitoring
- Opportunity Discovery
- Improved Analytics
- Enhanced Forecasting
- Value Tracking
- Faster Response
Testimonials
Director at the Responsible AI Institute
“Mr. Stambaugh was a key resource in setting up the Responsible AI practice at Suncor. His broad knowledge across the technology space, including AI, helped us bring together the multiple parties required to define and launch the Responsible AI program. I look forward to working with Mr. Stambaugh in the future as Responsible AI becomes a fundamental practice in any company looking to leverage AI in their business. Mr. Stambaugh is a rigorous, thoughtful and human-centric professional and I would welcome any opportunity to collaborate with him in the future.”
Director of Advanced Analytics / AI at a Major North American Energy Firm
“Personally I really appreciated Mr. Stambaugh’s help in standing up our Analytics Centre of Excellence and executing on a program to deliver and improve. His experience in this area helped avoid several possible pitfalls and I valued his wise council. I would definitely recommend utilizing Mr. Stambaugh’s skills and experience in any similar initiatives.”
International Information Security Professional
“I’ve collaborated with Mr. Stambaugh several times over the last six years to transform the information security programs of large enterprise customers. One of the key things that needs to take place to begin this transformation is to gain agreement among stakeholders who often have wildly different viewpoints. He excels at building this consensus with patience and persistence, always delivering successful outcomes.
Mr. Stambaugh has proven himself capable in all the roles I’ve seen him play – technology columnist, security analyst, project manager, technical architect, policy writer. Wherever Mr. Stambaugh goes, positive results follow. I would be thrilled to work together yet again.”
AI Leader in the Energy Sector
“Mr. Stambaugh is a great resource to have on any project team. He helped the team align on various initiatives such as governance and the charter for our Centre of Excellence to name a few. He is a very good listener and is able to communicate across various personas that come with different skillsets and ideas. Thank you Mr. Stambaugh!”
Telecommunications Leader
“Mr. Stambaugh is a person with great skills and deep expertise of modern business solutions! His skills in servant leadership has him building the capacity of others and enables them to perform at the highest degree. Furthermore, Mr. Stambaugh is dedicated, very capable team player and always works towards maximal customer satisfaction. He is not only a reliable and forward-thinking leader but leads by example which naturally generates enthusiasm and dedication from those around him. I could always depend on him to set the best possible example at Shaw Communications. He is truly remarkable and a one-of-a-kind person which I will always carry in my mind and heart in the highest esteem.”
More detailed achievements, references and testimonials are confidentially available to clients upon request.
Client Telephone Conference (CTC)
If you have any questions or if you would like to arrange a Client Telephone Conference (CTC) to discuss this particular Unique Consulting Service Proposition (UCSP) in more detail, please CLICK HERE.